
All data and processing on your account
Outerbounds deploys on your cloud account, so all data and processing stays under your governance. Outerbounds manages the infrastructure 24/7 through a stateless control plane, combining the benefits of a SOC2-certified fully managed service with the security and compliance of an on-prem solution.
End-to-end identity
Outerbounds integrates with your SSO provider, such as Google or Okta, making user management straightforward. Thanks to the integrated platform, the identity is managed consistently from prototype to production, including stages that go through CI/CD systems.
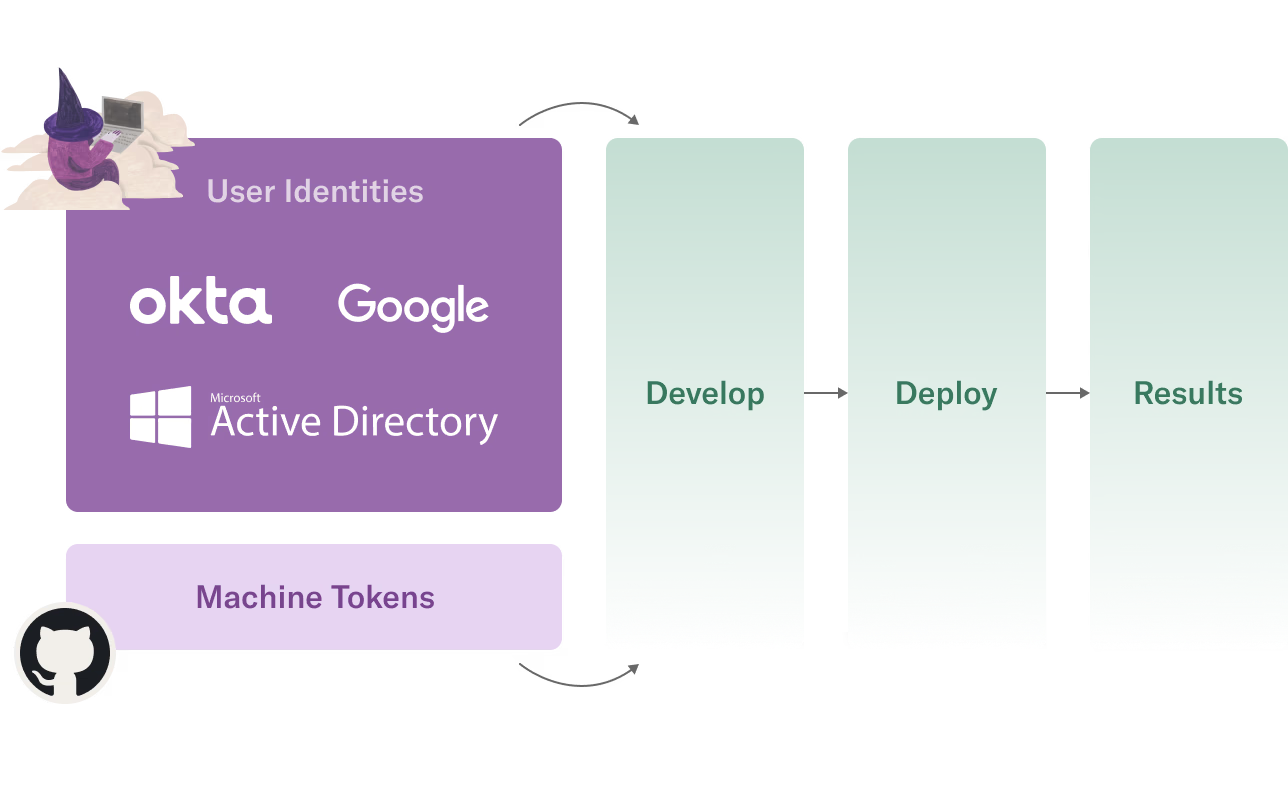

Bring your own policies
Outerbounds supports diverse AI, ML, and data projects. You can define specific outer bounds for each project, making sure they stay secure and compliant by default.
When using Outerbounds, users rely on your existing IAM roles, managed secrets, and other resources - all tracked in comprehensive audit logs - so you can integrate ML and AI in your enterprise confidently.