Scaling ML Operations at BlackCrow: From Months to Weeks with Outerbounds

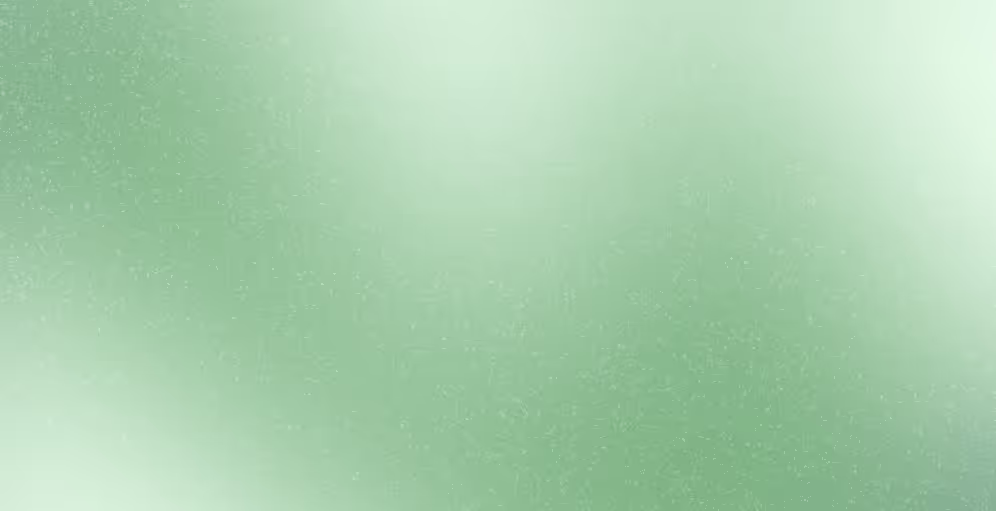

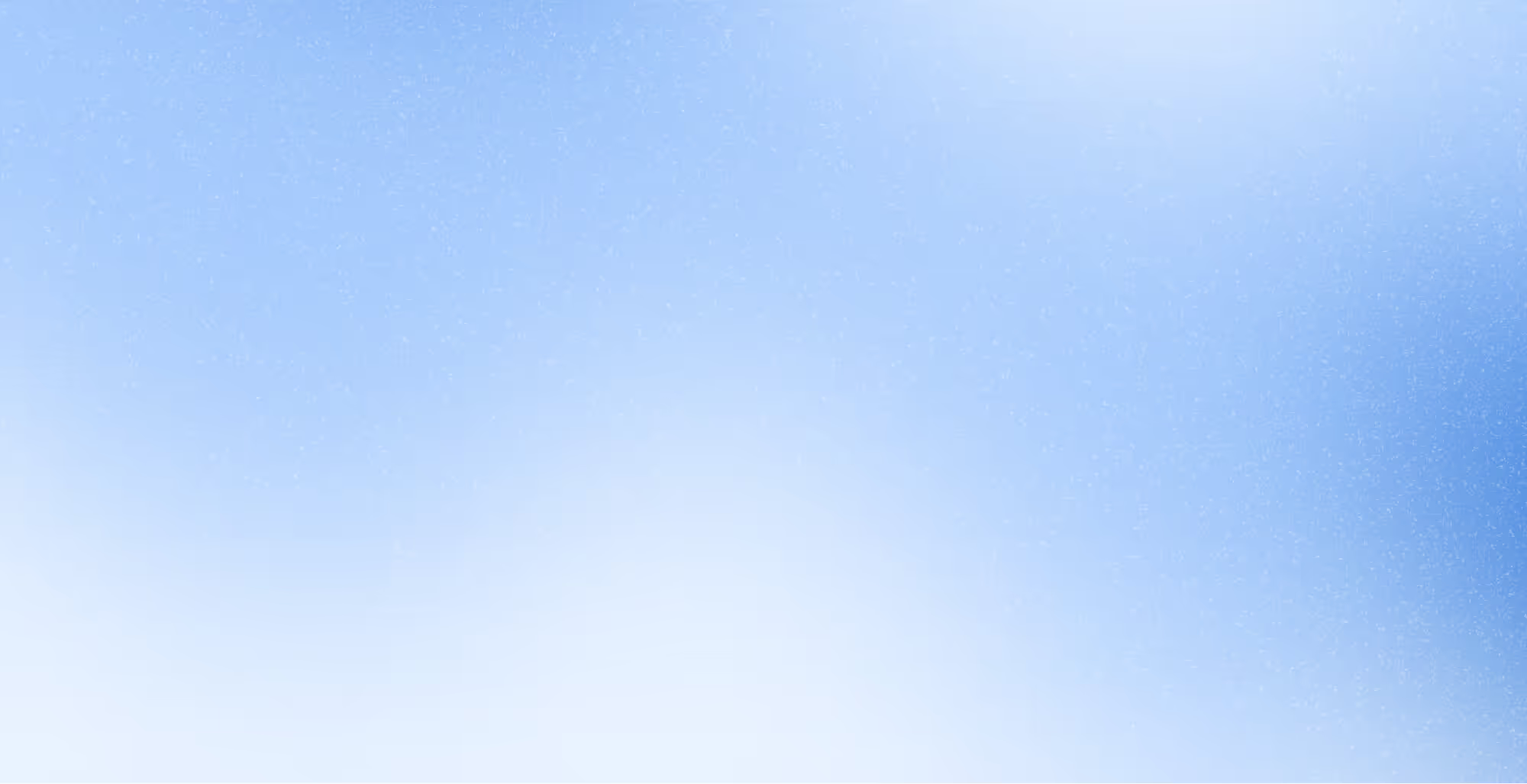
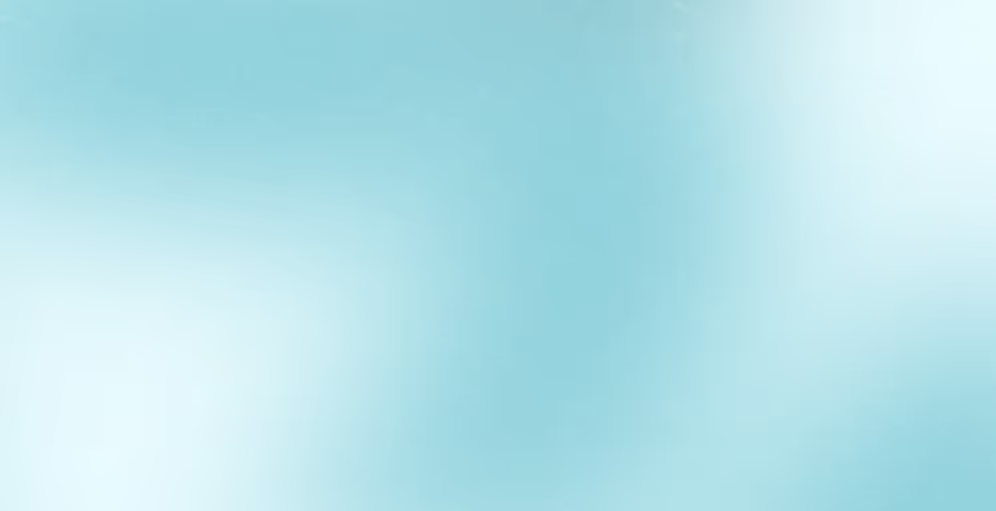
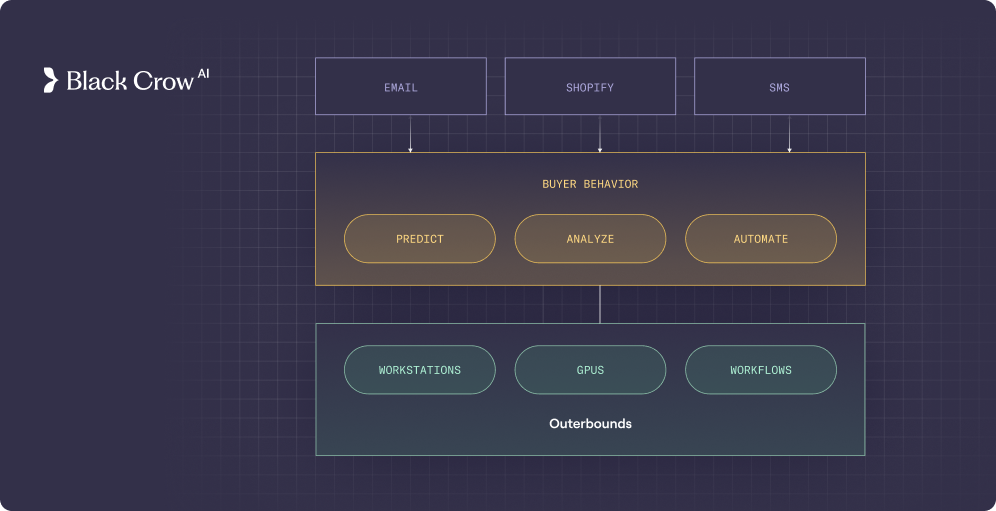

Deployment time dropped from 6 months to 2 months.
Achieved 4x faster deployment of machine learning models.
Increase in model autonomy: data scientists can now deploy models independently.
Black Crow AI is a cutting-edge AI platform focused on helping marketers optimize their ad spend, audience targeting, and overall marketing performance through advanced machine learning (ML) and AI-driven solutions. At the forefront of this effort is Camelia Hssaine, the Head of Data Science, who has been leading the data science team for over a year. Her responsibilities include managing data scientists, analysts, and ML engineers who are tasked with creating predictive models and scalable data systems to help Black Crow’s clients maximize their return on investment in digital marketing.
Before joining Black Crow, Camelia gained extensive experience at companies like Uber and Codecademy, where she built and scaled machine learning systems. Her journey from a mechanical engineering background to leading data science teams has been defined by her focus on solving complex, real-world problems through data. When she joined Black Crow, one of her first major initiatives was to overhaul the company’s approach to machine learning operations (MLOps) to make the team more agile, self-sufficient, and able to deliver high-quality ML models at scale.
At the time of Camelia’s arrival, Black Crow had a talented team of data scientists and engineers but lacked a cohesive ML platform that allowed them to easily develop, deploy, and monitor their models. This gap in infrastructure significantly slowed down the company’s ability to respond to market needs and build products quickly. As a result, finding a robust, scalable solution for their ML operations became Camelia’s top priority.
Manual, Lengthy, and High-Lift ML Deployment
The primary challenge Black Crow faced was the absence of an efficient ML operations platform. The data science team was responsible for building sophisticated models, but without an automated and scalable system in place, deployment and productionizing these models were arduous and time-consuming. Each model deployment was a high-lift process that required significant manual intervention from both the data science and engineering teams.
Camelia described the situation as “high-lift,” noting that the process of moving from model development to deployment could take 3-6 months under the best circumstances. In one extreme case, it took Black Crow 6 months to deploy a model because they lacked the infrastructure to do it efficiently. "Our first model deployment took 6 months," she shared, highlighting the challenge of scaling with the existing setup.
A significant pain point was the dependency on software engineers to deploy the models. Because data scientists at Black Crow were primarily focused on model development, they lacked the software engineering expertise required for model deployment. As a result, there was a lack of ownership over the end-to-end process, creating bottlenecks and slowing down productivity.
Another major issue was the lack of visibility and monitoring throughout the ML pipeline. When errors or bugs occurred, it was difficult for the team to pinpoint the exact location or cause of the issue, leading to extended delays in resolving problems. "Before, deployment was always the hardest part. Outerbounds made it so approachable and easy to use," Camelia remarked, explaining how this bottleneck was one of the most frustrating aspects of their workflow.
Additionally, the team had limited insight into the cost structure of running ML models, which made it challenging to justify scaling up their operations. With no clear visibility into compute and storage costs, data scientists struggled to make a compelling case for further investment in ML infrastructure.
Why Outerbounds Was the Right Fit
After evaluating various MLOps platforms, Camelia and her team ultimately chose Outerbounds, the commercial platform built on top of Metaflow, Netflix’s open-source machine learning framework. Outerbounds offered an intuitive UI and advanced features that made it easier for data scientists to develop, deploy, and monitor their models without relying on software engineers.
“Metaflow was an easy choice...it became the first marker of what our ML platform could look like,” Camelia said, reflecting on the decision-making process. The team had experimented with Metaflow in the past but hadn’t fully committed to it due to some initial limitations. However, with the introduction of Outerbounds, which added more robust features and a user-friendly interface, the platform became a clear winner.
Camelia emphasized that the ease of setup and integration was a major deciding factor. The goal was to give data scientists full autonomy over the entire machine learning lifecycle, from development to deployment, without needing to loop in software engineers for every deployment task. "Outerbounds was like Metaflow, but with a ‘plus plus’—it made everything easier," she added, referencing the additional value that Outerbounds brought compared to just using Metaflow.
Key features of Outerbounds that stood out for Black Crow included:
- Workstations: These provided the team with scalable, cloud-based environments to experiment with their models at a faster pace. "The workstations we use a lot," Camelia noted, adding that it was crucial for their development and testing phases.
- Versioning: Given that multiple data scientists were often working on different models simultaneously, the versioning capabilities helped manage this complexity. "Versioning is super helpful, just because we have many people who work on different models at the same time," Camelia explained.
- Experimentation Tracking: This feature enabled the team to track the progress of various model iterations, which sped up the process of refining and optimizing models. "The ability to experiment and track models has made iteration so much faster," she said.
The UI also played a big role in the decision. “The UI made the entire process so much more intuitive, especially for data scientists,” Camelia said. By offering a visual interface that provided insights into the entire machine learning flow, Outerbounds made it easier for the team to understand and manage their workflows.
Accelerating Deployment and Scaling ML Operations
The impact of adopting Outerbounds was immediate and transformative for Black Crow. The most significant improvement was in deployment time. With the old system, model deployment could take anywhere from 3 to 9 months, depending on the complexity of the model. With Outerbounds, the deployment time for even complex models was reduced to 1-2 months.
"We went from spending nine months on a model deployment to just 1-2 months with Outerbounds," Camelia highlighted, underscoring how much time the platform saved the team. This dramatic improvement allowed Black Crow to scale its ML operations and deploy more models in less time, which was critical for delivering timely solutions to clients.
Additionally, the time to resolve bugs and errors was drastically reduced. "Our time to resolve issues has expedited drastically because of the visibility Outerbounds gives us," Camelia shared. The team could quickly identify where an issue occurred in the workflow and address it in real-time rather than spending days or weeks troubleshooting.
Another significant benefit was the autonomy it gave to the data science team. Previously, data scientists had to rely on software engineers to deploy models, but now they could do it independently. "Outerbounds allows our data scientists to deploy models independently without relying on engineers," Camelia explained. This increased ownership and freed the engineering team to focus on other critical tasks.
Moreover, the platform provided greater visibility into the cost structure of running ML models. By offering insights into compute and storage costs, Outerbounds made it easier for the team to predict and manage expenses, enabling them to make data-driven decisions about scaling up their operations.
Outerbounds has fundamentally transformed how Black Crow approaches machine learning operations. The platform’s intuitive UI, powerful features like workstations and versioning, and the ability to empower data scientists to manage their own deployments have resulted in faster model deployment, increased autonomy, and more scalable ML workflows.
As Black Crow continues to grow, it is now able to deliver high-impact machine learning solutions to its clients in a fraction of the time. "The transition to Outerbounds was quick...we deployed a complex model in just 1-2 months," Camelia noted, encapsulating the overall success of the implementation.
Looking forward, Black Crow AI plans to expand its use of Outerbounds, particularly as they delve deeper into Generative AI and explore new ways to integrate advanced AI models into their platform. For Camelia and her team, Outerbounds has become an indispensable part of their machine learning toolkit.
Start building today
Join our office hours for a live demo! Whether you're curious about Outerbounds or have specific questions - nothing is off limits.
.png)