Scaling Global Financial Inclusion with Real-Time Machine Learning Using Outerbounds

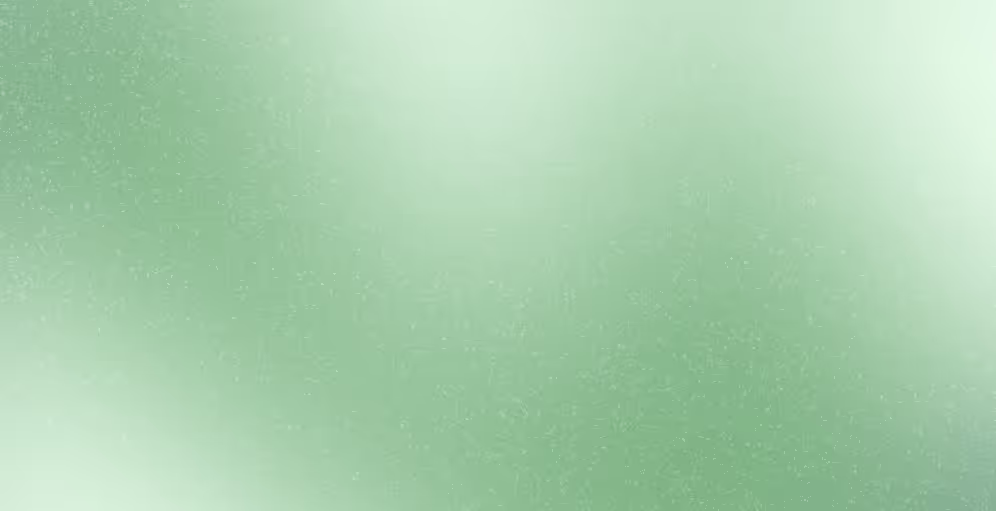

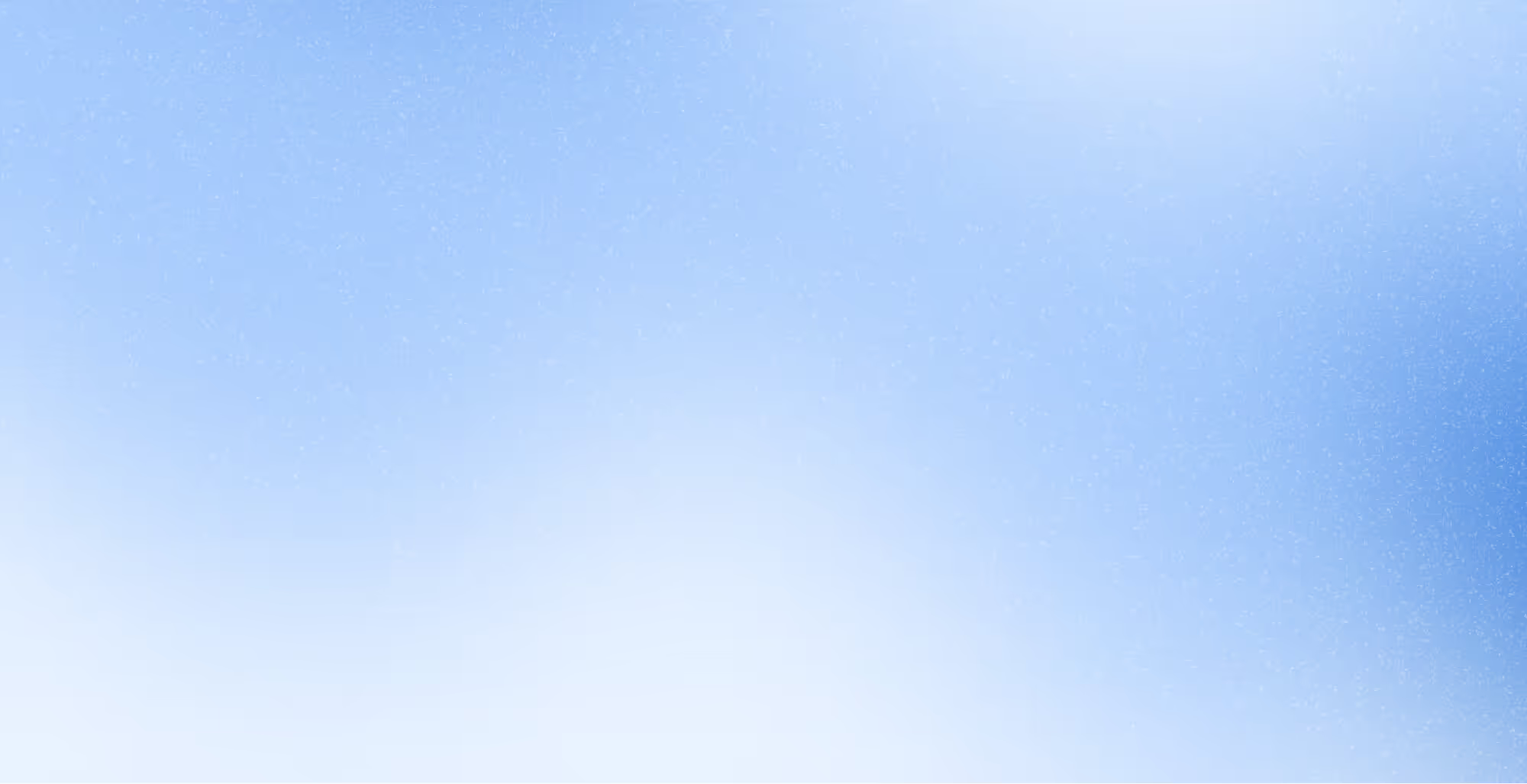
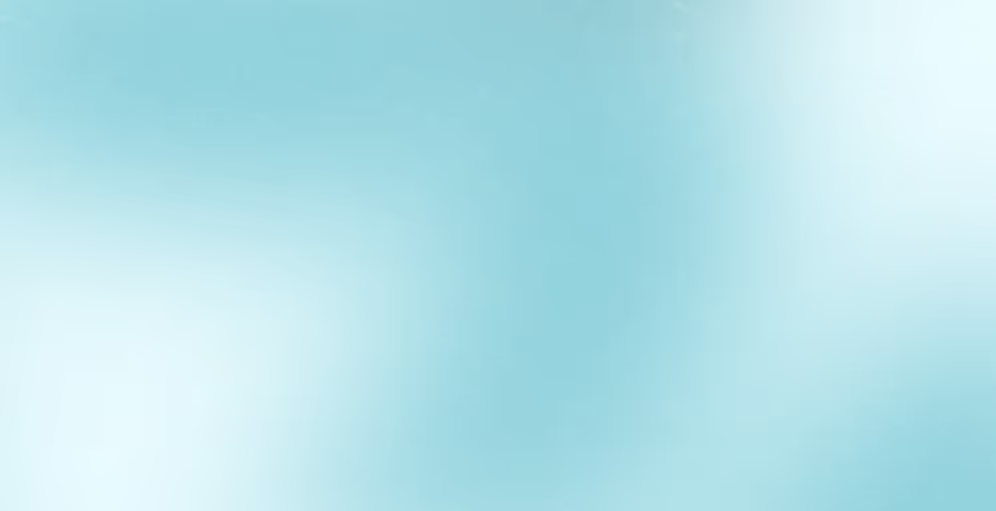
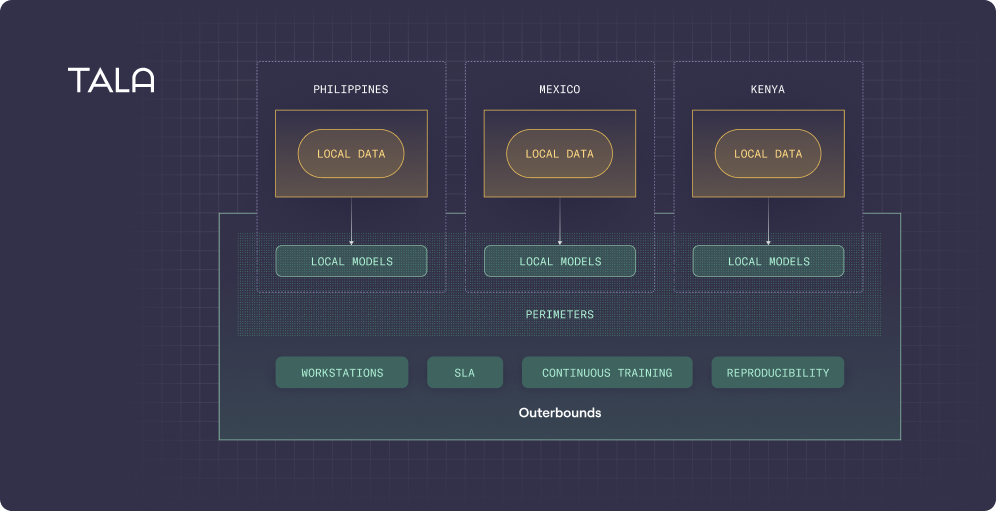
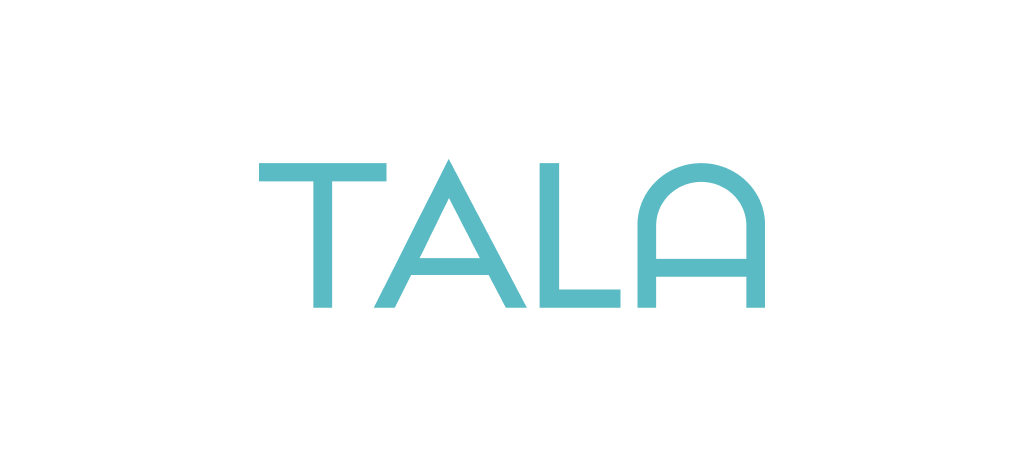
Increase in annual model deployment frequency.
Reduction: onboarding new data scientists now takes days instead of months.
Model retraining time decreased from months to days, boosting operational efficiency.
Tala is a financial services platform and micro-lender operating in the Philippines, Mexico, and Kenya, serving historically underserved populations. As a for-profit company dedicated to social impact since its founding, Tala’s business model prioritizes scalability and sustainability while driving financial inclusion. Will High, the Head of Data Science and Machine Learning Engineering, leads the team that manages real-time credit approval models, ensuring decisions are made in seconds.
“Shivani Siroya, our founder, made an intentional choice early on to pursue a for-profit model, believing it would allow us to build a generational company that could ultimately serve more people than we ever could as a nonprofit," " Will explains. "To achieve the scale we’re aiming for, we leverage nontraditional data and innovative DS-based underwriting, performing real-time credit assessments on Android devices," which dominate mobile usage in Tala's markets. However, the rapid pace and scalability needed in emerging markets demands robust infrastructure to automate machine learning pipelines, and that’s where Outerbounds comes in.
Challenges Faced Before Outerbounds
Before adopting Outerbounds, Tala faced considerable challenges with their machine learning infrastructure. Will’s team had tried Kubeflow and Airflow, but found them to be burdensome.
"Kubeflow was hard to manage. It required too much configuration and too many low-level commands," says Will. Their machine learning workflows were slow, cumbersome, and time-consuming, with manual retraining of models in Jupyter notebooks taking months. Any attempt to update a model would often lead to broken code, leading to significant delays. This created a bottleneck in the deployment of new models and added to the overhead of managing the infrastructure.
The lack of automation in model retraining and deployment became a major roadblock. In a business where creditworthiness is calculated in real-time, maintaining accurate, up-to-date models is mission-critical.
"We used to have engineers set up EC2 instances and run Jupyter servers manually, which would take months to onboard a new data scientist," Will notes.
The existing system wasn't scalable, making it nearly impossible for the team to handle growing data volumes and increased demand from the expanding customer base. This inefficiency drove Tala to search for a better solution.
The Search for a Solution
Tala was already familiar with Metaflow, an open-source framework that had been adopted at Netflix to scale machine learning projects. Will himself was an early adopter of Metaflow during his time at Netflix. As a natural next step, he and his team evaluated both the open-source version of Metaflow and other platforms like MLflow and Kubeflow.
"I’ve been using Metaflow since before it was open-sourced, but we needed more—something that would give us the scalability and compliance we required," says Will.
After testing the open-source version, Will's team realized they needed a more managed solution. Enter Outerbounds, which provided an enhanced version of Metaflow tailored for enterprises. The SLA-backed services, intuitive user interface, and compliance features were pivotal in Tala’s decision to choose Outerbounds.
"Outerbounds offered a UI that we loved and a strong SLA, which was critical for our operations. The automation it enabled was a game-changer," Will recalls.
A Leap in Efficiency and Scalability
Once Tala onboarded Outerbounds, the difference was night and day. The platform enabled full automation of their model retraining processes, reducing manual intervention and increasing overall efficiency. The team could now ensure that models were continuously retrained with fresh data.
"We used to spend months fixing broken notebooks; now, with Outerbounds, it's all automated. The agility we gained is incredible," says Will. This automation was crucial in allowing the team to handle the vast amounts of data they process daily, especially as they expand to new markets.
Outerbounds also improved the onboarding process for new data scientists. Before, it took months for a new hire to get set up with infrastructure, but now it takes just days. The seamless integration with existing cloud environments, like AWS, and the compliance features built into the platform allowed Tala to meet industry-specific regulatory standards.
"Our onboarding process for new data scientists went from months to just days. That’s a huge win for us," Will emphasizes.
The increase in model deployment velocity directly impacted Tala's business. They went from deploying only a few models a year to over five times that amount, leading to more accurate credit decisions and better service for their customers. In addition, the UI provided by Outerbounds helped the team monitor workflows in real-time, making it easier to manage and debug when necessary.
"We now have five times the model deployments we had before, and the impact on our business is clear—we’re reaching more people, faster," adds Will.
Another significant advantage has been reproducibility. Tala’s data science team can revisit any model run from years past, ensuring compliance and helping them audit decisions if needed. This feature was critical for an industry as tightly regulated as financial services.
"Reproducibility is the sleeper feature. You don’t realize how important it is until you need it. Outerbounds has made it easy to access every run and artifact from the past two years," Will explains.
By adopting Outerbounds, Tala was able to:
- Automate model retraining, eliminating the need for manual intervention and reducing time from months to seconds.
- Increase model deployment frequency by 500%, driving real-time credit decision-making and fraud detection.
- Reduce onboarding time from months to days, making it easier for new data scientists to contribute to the team’s goals.
- Improve reproducibility and compliance, ensuring that all model artifacts could be revisited as needed.
In the future, Tala plans to further leverage Outerbounds’ capabilities, including scaling their operations to new countries and exploring AI-driven user experiences with the help of GPU-powered inference. The foundation laid by Outerbounds has positioned Tala for long-term growth and success in the financial services sector while furthering the company’s mission of financial inclusion.
"We are building something truly scalable, and Outerbounds has been critical in helping us achieve that," concludes Will.
Start building today
Join our office hours for a live demo! Whether you're curious about Outerbounds or have specific questions - nothing is off limits.
.png)