Revolutionizing Ecosystem Analytics and Restoration with Metaflow

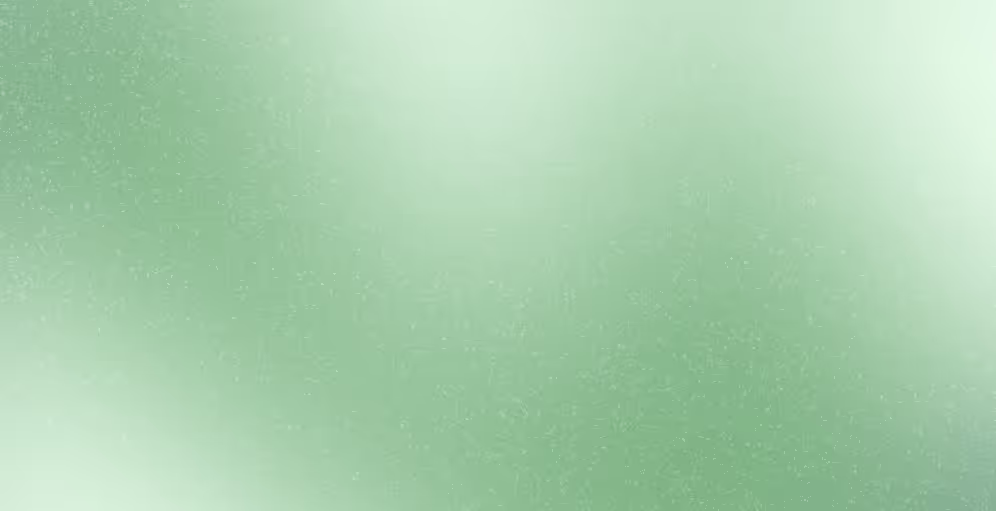

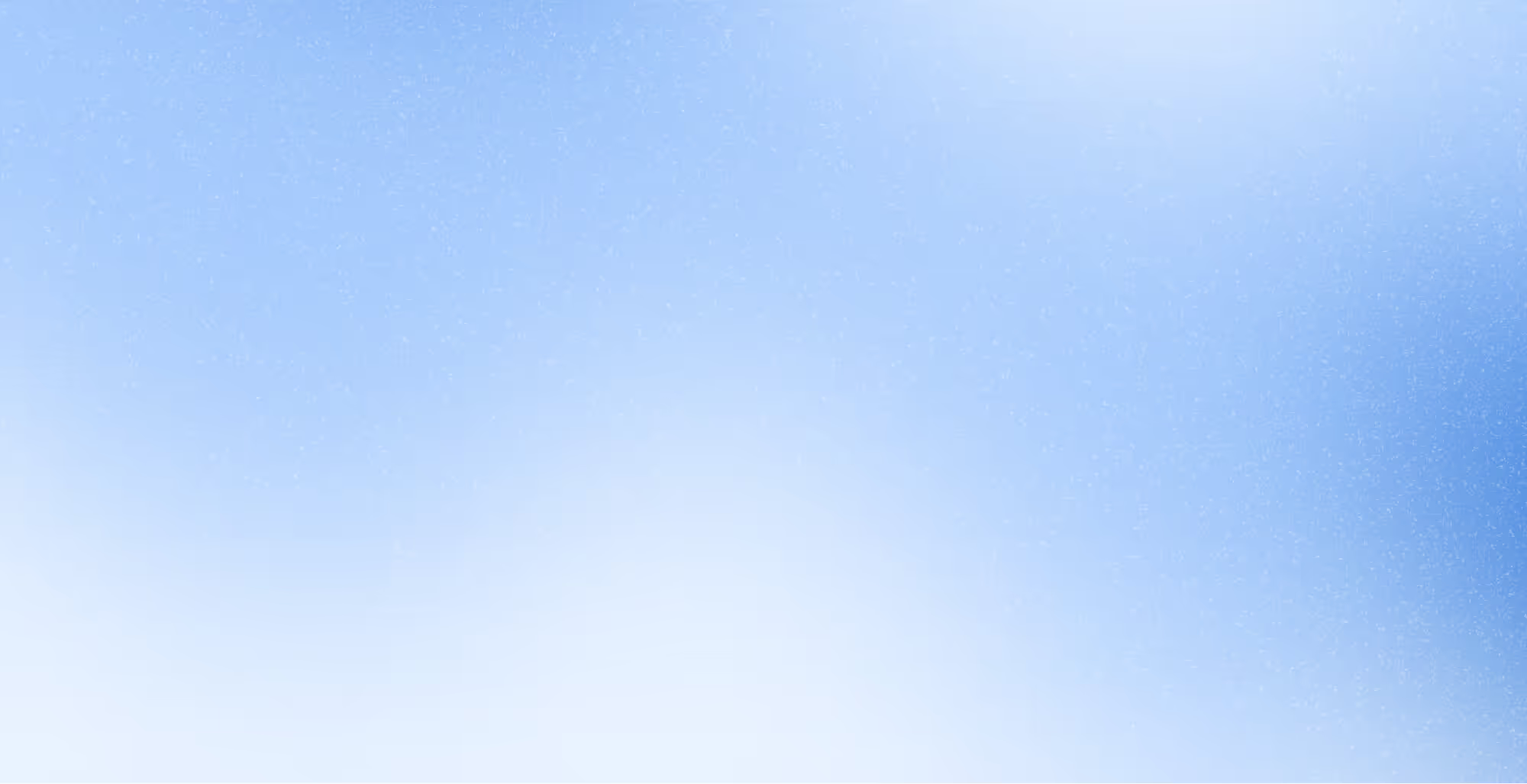
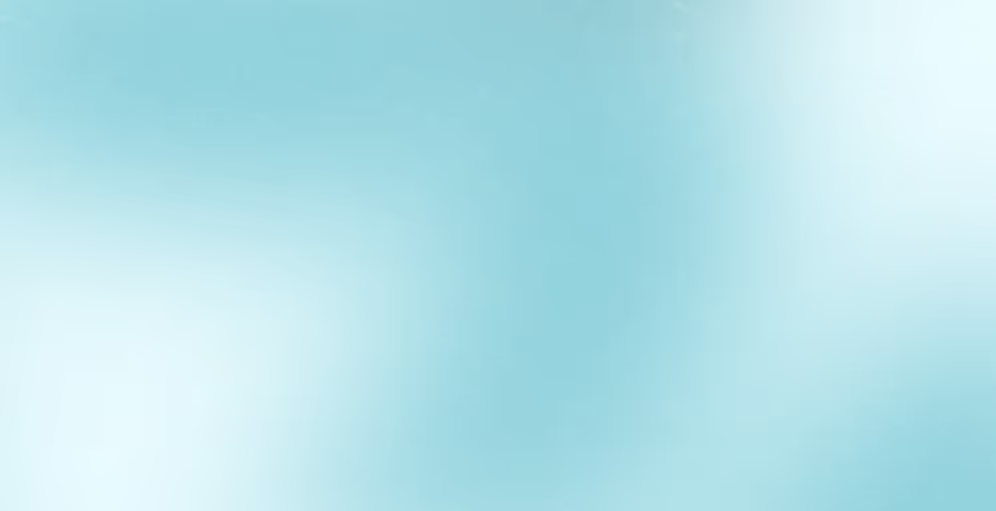
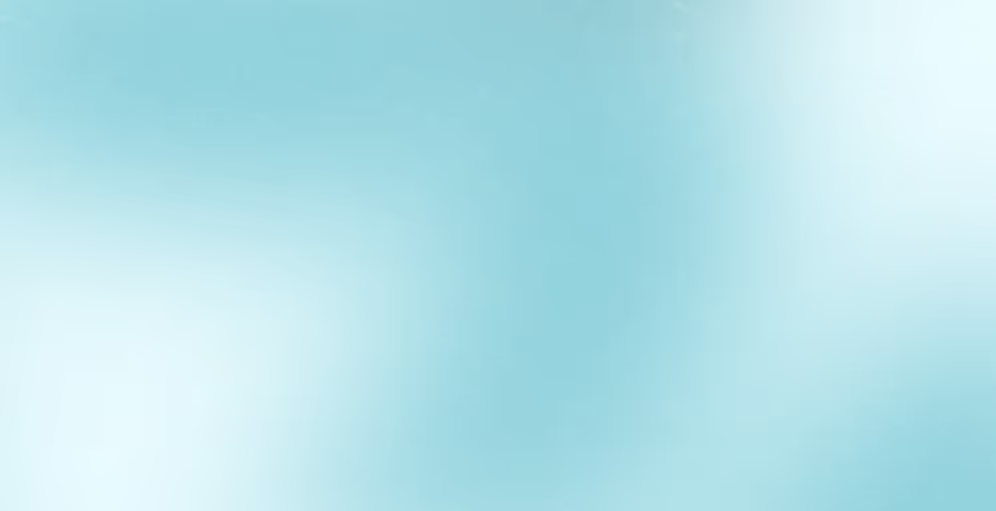

Reduction in manual artifact tracking – Prior manual tracking eliminated with Metaflow's artifact management.
Faster onboarding – New hires navigate machine learning workflows quicker with Metaflow’s structured process.
Reduction in manual debugging time – Faster issue resolution through shared artifact inspection and resumable workflows.
Dendra Systems, an organization at the forefront of ecosystem restoration, uses cutting-edge drone technology to plant millions of trees and monitor ecosystems worldwide. Dendra’s mission is to tackle global environmental challenges by restoring damaged ecosystems, using fleets of drones to reseed and provide in-depth ecosystem analytics. This includes identifying species, tracking invasive plants, and assessing overall ecological health. At the heart of Dendra’s technological innovation is machine learning, which enables their teams to analyze aerial imagery captured by drones.
Richard Decal, an ML lead scientist at Dendra, has been with the company for over four years and played a pivotal role in developing its machine learning infrastructure. Richard’s focus is on species identification through computer vision models, where the challenge lies in analyzing vast datasets from aerial images and deriving meaningful insights. Under his leadership, the ML team transitioned to Metaflow, a decision that significantly improved the team’s productivity and scalability.
The Challenges Before Metaflow: Fragmented Workflows and Bottlenecks
Before adopting Metaflow, Dendra Systems faced several critical challenges in their ML workflows. Like many early-stage ML environments, the team operated with a collection of disjointed Python scripts. These scripts ran independently, and managing the process of preparing data, training models, and tracking outputs was both cumbersome and error-prone.
One of the most significant issues Richard’s team encountered was related to manual artifact tracking. "We were using S3 to store our artifacts, and it became increasingly difficult to manage," said Richard. "We had to worry about naming conventions, overwriting artifacts, and keeping track of the lineage of experiments."
This resulted in a situation where team members often spent time diagnosing issues that stemmed from inconsistencies in dependencies and incompatible versions across their stack. "I remember one time we had an intern with a different version of Pandas," Richard explained. "This caused errors when trying to load artifacts because the versions were incompatible."
Another key pain point involved collaboration across the team. Since their early system didn’t facilitate seamless artifact sharing or workflow tracking, team members had to rely on direct communication and manual intervention to share experiment results or troubleshoot issues. As Richard pointed out, “In the past, if a process failed for one team member, another couldn’t simply resume from that point without restarting the entire process from scratch.”
Dendra also struggled with deployment and scaling challenges. The team needed a tool that could easily shift between local and cloud environments, something that their previous systems, such as Kedro and MLflow, could not handle effectively. Furthermore, manually cataloging parameters and experimenting with different models became a logistical nightmare, hampering both productivity and experimentation.
Finding the Right Tool: Metaflow’s Key Role in Optimizing ML Workflows
After evaluating various solutions, Richard’s team discovered Metaflow, an open-source platform designed to handle complex data science workflows. Dendra needed a tool that could not only manage artifacts but also integrate seamlessly into their cloud-native environment on AWS. Metaflow’s promise of tracking and managing artifacts in a transparent, automated manner was a key differentiator that stood out.
Why Metaflow?
Richard highlighted several specific requirements that led Dendra to Metaflow:
- Artifact Tracking: “Artifact tracking was one of the biggest problems we had. Metaflow’s ability to automatically handle this was a game changer for us,” Richard said.
- Resumable Workflows: The team wanted the ability to resume runs when a job failed, without needing to restart everything from scratch. “If something goes wrong during a long-running process, the ability to resume the flow was critical for us.”
- Cloud Integration with AWS: Dendra needed a tool that could operate in their AWS-native infrastructure. Metaflow’s cloud-agnostic capabilities and tight integration with AWS fit this requirement perfectly.
- Developer-Friendly Experience: The Metaflow platform provides a clean developer experience, minimizing the need for manual interventions. “It just made the whole process of tracking and running experiments seamless,” Richard said.
While Metaflow did impose some structural requirements, such as the necessity to define workflows as a DAG, the advantages it brought far outweighed this limitation. As Richard noted, “It constrains you to a certain way of doing things, and that’s a methodology that we prefer. It enforces consistency, and that consistency is what makes things easier to scale.”
Post-Metaflow: Streamlined Workflows and Enhanced Collaboration
Since adopting Metaflow, Dendra’s machine learning processes have transformed, significantly enhancing both their productivity and scalability. What used to be a patchwork of scripts is now an organized, repeatable and powerful workflow that is easy to manage and debug.
One of the most significant improvements has been in collaboration across the team. With Metaflow’s shared environment, multiple team members can inspect a flow’s artifacts in real time without re-executing entire runs. "Before Metaflow, if a process failed for one person, another person would have to restart the job," Richard explained. "Now, with Metaflow, anyone can dive in and troubleshoot a problem by inspecting the flow’s logs and artifacts."
Onboarding New Team Members
Metaflow’s structured environment has also drastically improved onboarding times for new hires. Richard pointed out that learning Metaflow’s workflow is a one-time cost, after which new team members can easily replicate and modify existing flows. "In the past, the overhead of understanding all the scripts and dependencies was high," he said. "Now, with Metaflow, onboarding is significantly faster because everything is laid out clearly in the flow spec."
Zero Manual Artifact Tracking
The most significant time savings have come from eliminating manual artifact tracking. Richard mentioned, "Tracking and managing artifacts used to be a big part of the process, and it’s basically gone to zero now. Metaflow handles all of that for us, so we can focus on developing and improving our models."
Dendra Systems has seen substantial improvements since transitioning to Metaflow:
- Enhanced Productivity: Manual artifact tracking has been fully automated, reducing operational overhead and allowing the team to focus on core ML tasks.
- Improved Collaboration: Team members can now collaborate seamlessly by inspecting and debugging workflows in real-time, without restarting jobs.
- Scalability: Metaflow’s robust workflow management has enabled Dendra to scale their machine learning operations across a fleet of drones, analyzing millions of data points efficiently.
Metaflow has become an integral part of Dendra’s ecosystem analytics efforts, streamlining their workflow and positioning the team for future growth in ecosystem restoration. Looking ahead, Richard and his team are excited to expand their use of Metaflow’s capabilities further to optimize their machine learning models and continue innovating in the field of ecosystem restoration.
Start building today
Join our office hours for a live demo! Whether you're curious about Outerbounds or have specific questions - nothing is off limits.
.png)