Empowering Grid Optimization with Metaflow: Equilibrium Energy’s Success in AI-Driven Energy Management
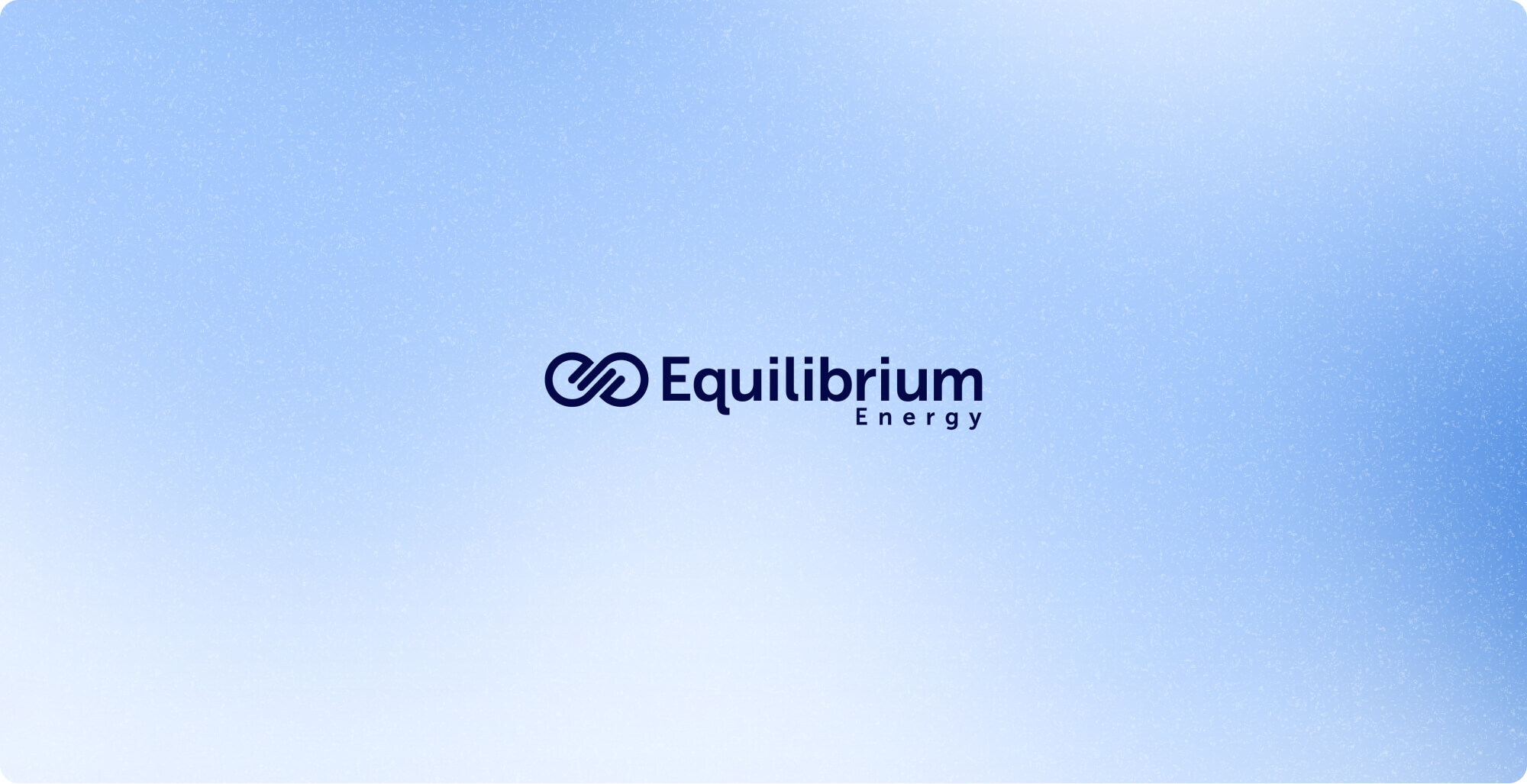
Onboarding success: new data scientists deploy models within 1-2 days of joining.
Sharing modules across teams prevents siloed workflows.
Continuous expansion of Metaflow use cases across inference and backtesting workflows.
Equilibrium Energy is at the forefront of AI-driven power management, specializing in optimizing grid-connected battery operations for energy trading. The company leverages cutting-edge artificial intelligence to forecast energy prices and manage large-scale grid batteries. Kevin McEntee, VP of Engineering at Equilibrium Energy, brings a wealth of experience from his time at Netflix and other Silicon Valley tech companies, contributing to the company’s mission of achieving climate impact through AI innovations.
“I joined EQ out of motivation to have climate impact and work on interesting AI problems,” McEntee explains. This combination of passion for climate tech and artificial intelligence defines Equilibrium Energy's approach, and their strategic use of Metaflow plays a central role in their success.
Challenges Before Metaflow
Prior to Kevin’s arrival, Equilibrium Energy was already using Metaflow for their AI operations. However, before Metaflow, the company relied on a homegrown solution for managing machine learning workflows. This approach posed challenges typical of many AI startups: a lack of standardization, fragmented workflows, and time-consuming efforts spent building custom tools rather than focusing on their core business.
“Why would we try to do our own thing when there's this great community we could tap into and make use of it?” says McEntee, referring to Metaflow’s open-source community.
The homegrown solution lacked the flexibility, speed, and scalability that a fast-growing startup required. As a small team focused on optimizing grid-connected battery operations, Equilibrium Energy needed a reliable and scalable framework for model training, inference, and backtesting.
The key pain points they faced were:
- Time-consuming custom workflows: Building in-house tools detracted from focusing on differentiating AI models.
- Siloed team efforts: Without a common platform, collaboration between data scientists and engineers was limited.
- Slow iteration cycles: Testing hypotheses and iterating on models took too long due to manual processes.
Why Metaflow?
When Kevin joined, the team was already using Metaflow, an open-source framework originally developed at Netflix. Metaflow was chosen to replace their homegrown solution due to its robustness, active development, and growing community support.
Metaflow allowed the company to focus on what mattered: their AI models. Its core capabilities offered a solution to their most pressing issues:
- Streamlined Workflow Management: By providing a uniform structure for building machine learning workflows, Metaflow eliminated the need for homegrown alternatives.
- Seamless Collaboration: Engineers and data scientists could work together more efficiently, thanks to Metaflow’s consistent environment across different projects.
- Speed and Iteration: With Metaflow’s support for features like checkpointing, the team could test hypotheses more rapidly. “Checkpointing allows us to iterate much faster, especially on the last steps of a multi-step flow,” explains McEntee.
Additionally, the ability to modularize workflows gave them a flexible way to share components across different teams. As McEntee describes, "The kind of modularization we're able to create and use in Metaflow helps us with sharing across different teams."
Metaflow in Action
At Equilibrium Energy, Metaflow is used across various workflows, ranging from model training to inference and scenario backtesting. These workflows are key to the company’s battery operations. For example, they rely on time series forecasting to predict energy prices, which drives decisions on when and how to charge or discharge batteries to optimize profits.
“We use our software to manage large grid-connected batteries and optimize them for energy trading,” says McEntee.
The flexibility of Metaflow allows the team to integrate it beyond traditional machine learning use cases. One significant example is its use in backtesting workflows, where they simulate battery operations over previous years to refine and improve their strategies. "We’ve expanded our use of Metaflow to use it as a runtime model for scenario backtesting," McEntee notes, highlighting how they are finding new ways to leverage the platform.
This expanding use of Metaflow demonstrates its flexibility and scalability. Equilibrium Energy no longer sees it as just a tool for model training but as a general scientific workflow engine capable of supporting broader business operations.
Results and Improvements with Metaflow
The impact of adopting Metaflow is evident in several key metrics. One of the most significant improvements is onboarding speed for new data scientists. When a new hire joins, they are expected to run models on their first or second day, thanks to the simplicity and user-friendliness of the Metaflow environment.
“We have a goal that a new data scientist starts running a model on their first or second day,” says McEntee. This is a marked improvement from the longer onboarding times common with other tools and environments.
Another area where Metaflow shines is in operational efficiency. By reducing the fragmentation in workflows and enabling the reuse of modules across teams, Equilibrium Energy avoids the pitfalls of duplicated work and inconsistent processes. This also helps reduce errors and makes debugging easier, as flows are standardized across the company.
If Equilibrium Energy hadn’t adopted Metaflow, McEntee believes their teams would be siloed, each running their own version of workflows, making collaboration and progress difficult. “If we didn’t have Metaflow, probably every science and ML pairing team would be doing their own thing,” he explains. Metaflow’s modular and consistent framework provides the necessary cohesion for a unified approach to AI and machine learning.
Adopting Metaflow
By adopting Metaflow, Equilibrium Energy has significantly improved the speed and efficiency of its machine learning workflows. From faster onboarding of new data scientists to the reuse of workflow components across teams, Metaflow has become the backbone of their AI-driven energy trading operations.
“Metaflow helps us move fast on testing hypotheses,” McEntee emphasizes, reinforcing the importance of speed and flexibility in their fast-paced industry.
Through its seamless integration with AWS and Databricks, the company benefits from a flexible, cloud-agnostic infrastructure that supports their mission to optimize grid-connected battery operations. As Equilibrium Energy continues to innovate and expand its AI capabilities, Metaflow will remain a critical tool for scaling their operations and driving further efficiencies across their workflows.
Equilibrium Energy is committed to deepening its use of Metaflow as their business grows. They plan to continue exploring more advanced features, including further automation of backtesting workflows and expanding Metaflow’s role in new AI-driven initiatives. Equilibrium Energy’s adoption of Metaflow has provided them with the tools necessary to accelerate their AI development, increase team collaboration, and optimize their core business operations in grid energy management. Through the use of Metaflow, they are positioned for continued success in the rapidly evolving energy landscape.