DeepTrust Secures Digital Communication with Outerbounds: From Days to Hours in ML Model Deployment

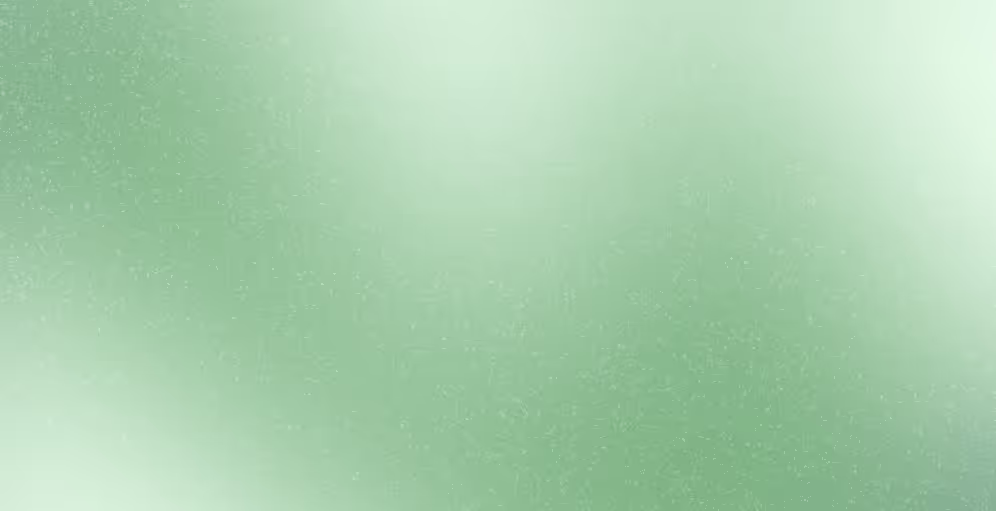

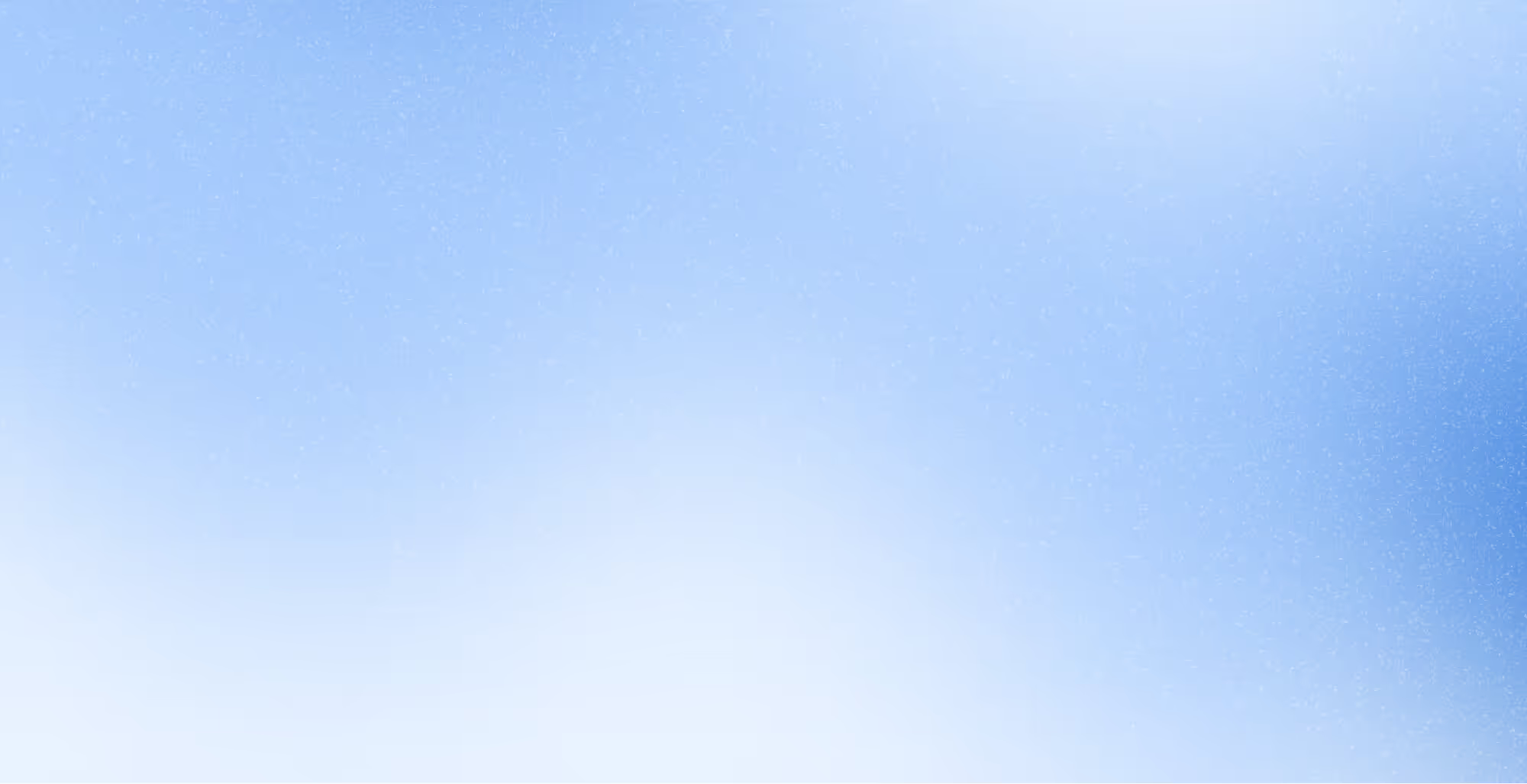
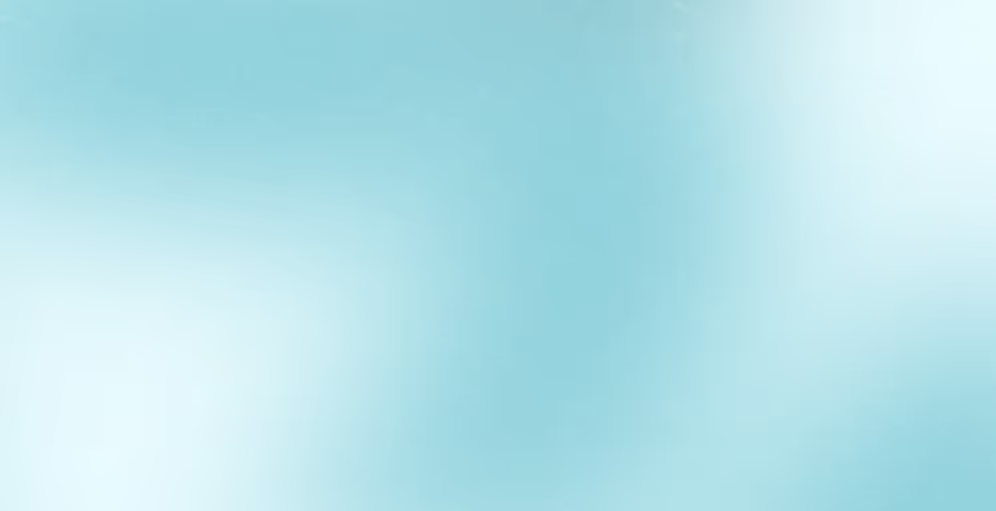
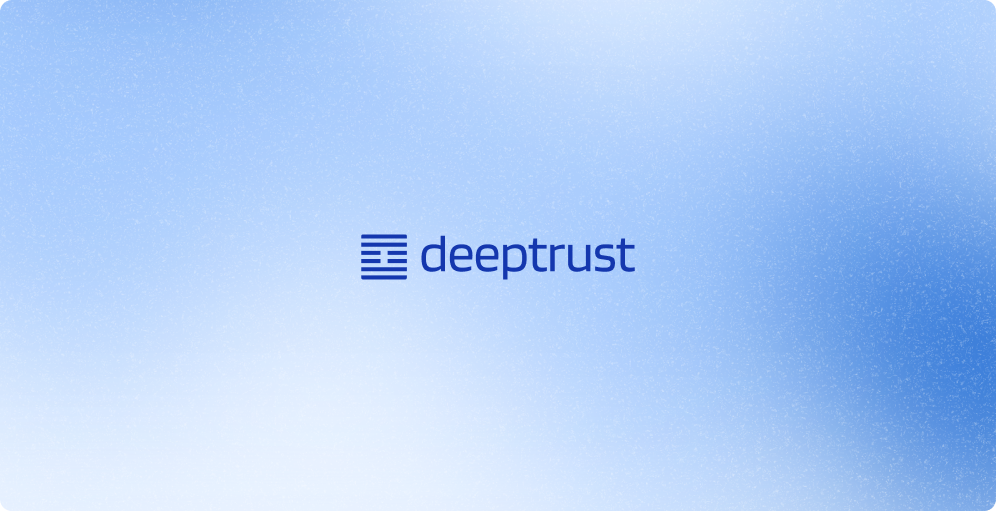

Reduction in model build time: Reduced model build time from 4 days to 6 hours.
Reduction in orchestration overhead: Moved from managing own ML infrastructure to using Outerbounds.
Faster deployment cycle: Reduced overall model deployment cycle by half.
DeepTrust is a cutting-edge startup focused on solving one of the most complex problems in the digital age: trust in online content. The company, founded by Aman Ibrahim, is on a mission to build a digital trust layer to combat social engineering attacks, voice phishing, and deepfakes. In an era where misinformation and manipulated content are rampant, DeepTrust’s goal is to provide organizations with the tools they need to secure communications and ensure the authenticity of what is seen and heard.
“Our ultimate ambition is to build a trust layer for the entire internet,” says Aman. The team at DeepTrust started by focusing on call security—using machine learning to help organizations protect themselves against social engineering attacks, voice phishing, and other threats in VoIP environments like Zoom and Microsoft Teams. This focus allows security teams to detect and prevent manipulation in real-time conversations.
However, despite their ambitious goals, DeepTrust faced significant challenges in scaling their machine learning operations efficiently, which led them to explore new solutions to accelerate their development pipeline.
The Challenge: Slow, Complex Machine Learning Infrastructure
Before adopting Outerbounds, DeepTrust faced multiple challenges in managing their machine learning infrastructure. Aman, who had previously worked at Cruise on the orchestration team, was no stranger to the difficulties of building scalable machine learning workflows. At Cruise, his team developed an in-house system similar to Metaflow, Netflix’s open-source machine learning platform, which required a substantial amount of manual effort to manage.
At DeepTrust, the team initially handled machine learning pipelines through a series of scripts that were scattered across different services. "We had multiple scripts running across different environments—one for ETL, another for model training, another for deployment," Aman explains. This fragmented setup resulted in inefficiencies, especially when it came to onboarding new engineers or trying to scale their machine learning operations. "It would have been very difficult to onboard someone to all these different technologies. They would need to understand not just our business logic, but the specific quirks of each system."
The process of building and deploying machine learning models was slow and manual. "Before Outerbounds, from data collection to a fully trained model, it would take us three to four days," Aman recalls. The delay posed a significant bottleneck in their operations, as speed was critical for developing new security features to detect evolving threats like deepfakes and voice phishing attacks.
Moreover, managing their own infrastructure required considerable overhead, with the team having to manually spin up containers, manage compute resources, and handle event triggers. “The overhead was slowing us down,” Aman said. “We didn’t want to be in the business of managing infrastructure; we wanted to focus on solving the trust problem.”
The Solution: Why DeepTrust Chose Outerbounds
Aman was already familiar with Metaflow and its capabilities from his time at Cruise, so exploring Outerbounds—an enhanced managed platform built on Metaflow—was a natural step. “The most exciting thing about Outerbounds was how it could accelerate us, especially as a small team,” Aman explains. “We didn’t want to worry about managing infrastructure or writing scripts to scale resources. We needed a solution that would let us focus on our core business.”
Several key factors influenced DeepTrust’s decision to adopt Outerbounds:
- Ease of Use and Documentation: Outerbounds builds on Metaflow’s user-friendly API and comprehensive documentation, making it easy for engineers to pick up. “The ease of consuming the syntax and the availability of clear documentation were key. We needed something that was simple for our team to adopt quickly,” Aman noted.
- End-to-End ML Management: One of the standout features for DeepTrust was the ability to manage the entire machine learning lifecycle within a single platform. From model training and deployment to artifact management and experiment tracking, Outerbounds provided all the essential tools. “Having everything in one place—event triggers, experiment tracking, managed artifacts—was a big deal for us,” said Aman.
- Flexibility: Outerbounds offered the flexibility to use custom Docker containers, PyTorch, and other tools that were vital for DeepTrust’s specific use cases. This was crucial as they needed a platform that could adapt to their unique machine learning needs, especially when fine-tuning language models for security contexts.
- Strong Support: Another factor that sealed the deal for DeepTrust was Outerbounds’ dedicated support team. “Support was a huge selling point. We knew if something went wrong, we’d have the backing of the Outerbounds team to fix it quickly,” said Aman. This level of support was particularly important for a startup operating on tight timelines.
With these requirements in mind, DeepTrust decided to adopt Outerbounds and leverage its managed services to streamline their machine learning workflows.
Implementation and Results: A 4-Day Process Reduced to 6 Hours
The transition to Outerbounds was seamless for DeepTrust. They implemented the platform via the AWS template provided by Outerbounds, which made the onboarding process quick and straightforward. "The onboarding experience was so smooth that it was almost validation in itself that we made the right decision," Aman said. “It was a matter of logging in, connecting our accounts, and then everything just worked.”
One of the most significant improvements was the reduction in time-to-deployment for machine learning models. Before implementing Outerbounds, the process of training a model from scratch took three to four days. Now, with Outerbounds’ automated event triggers and infrastructure management, that process has been reduced to 6 hours. "I just kick off the process and check back in six hours, and the model is trained. It used to take us days," Aman explained. This 85% reduction in model build time was a game-changer for DeepTrust, allowing them to iterate faster and respond more quickly to emerging security threats.
In addition to speeding up model training, DeepTrust also saw a 100% reduction in orchestration overhead. By using Outerbounds’ managed infrastructure, the team no longer had to manually manage containers or compute resources. “We don’t need to worry about spinning up infrastructure or handling event triggers ourselves anymore. It’s all taken care of,” said Aman.
Another key improvement was in the onboarding process for new engineers. Previously, onboarding required teaching new hires the intricacies of multiple different systems. Now, with Outerbounds, everything is consolidated into one platform, making it much easier for new team members to become productive quickly. "It’s much easier to onboard engineers now that we’re using one platform for everything," Aman noted.
DeepTrust’s decision to adopt Outerbounds has paid off in several measurable ways. By reducing the time-to-deployment for machine learning models from days to hours and eliminating the overhead of managing their own infrastructure, the team has been able to focus more on their core business of securing digital communications.
Key successes include:
- 85% reduction in model build time: From 3-4 days to 6 hours, allowing faster iteration and deployment.
- 100% reduction in orchestration overhead: Freed up the team to focus on business value rather than infrastructure management.
- Streamlined onboarding: Consolidated machine learning processes into one platform, making it easier for new engineers to get up to speed.
As DeepTrust continues to grow, they plan to leverage more of Outerbounds’ advanced features, including multi-cloud support and potential fine-tuning of models for specific industries like finance. Their success with Outerbounds has positioned them to be more agile and responsive in combating the digital threats of today—and tomorrow.
Start building today
Join our office hours for a live demo! Whether you're curious about Outerbounds or have specific questions - nothing is off limits.