Accelerating Imaging Workflows with Metaflow at GE Healthcare

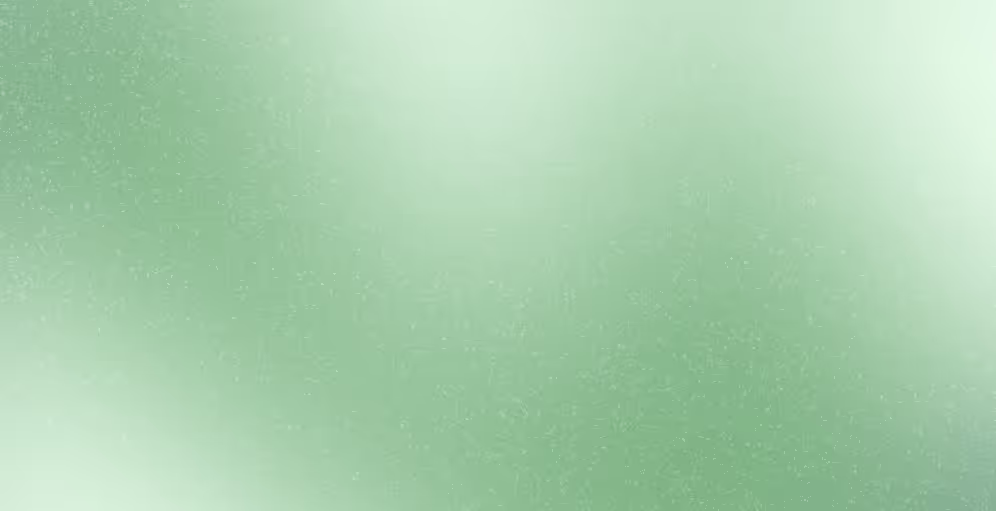

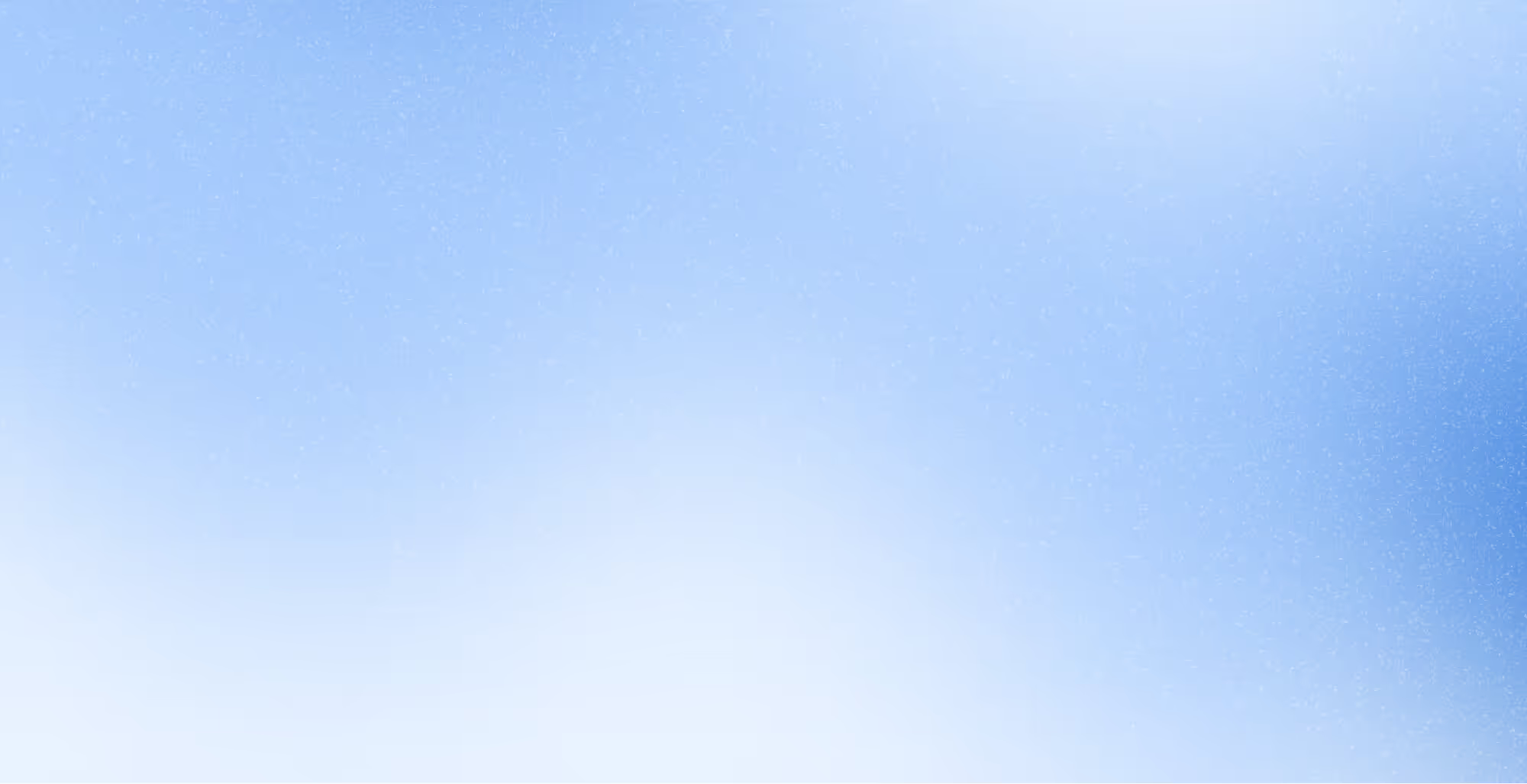
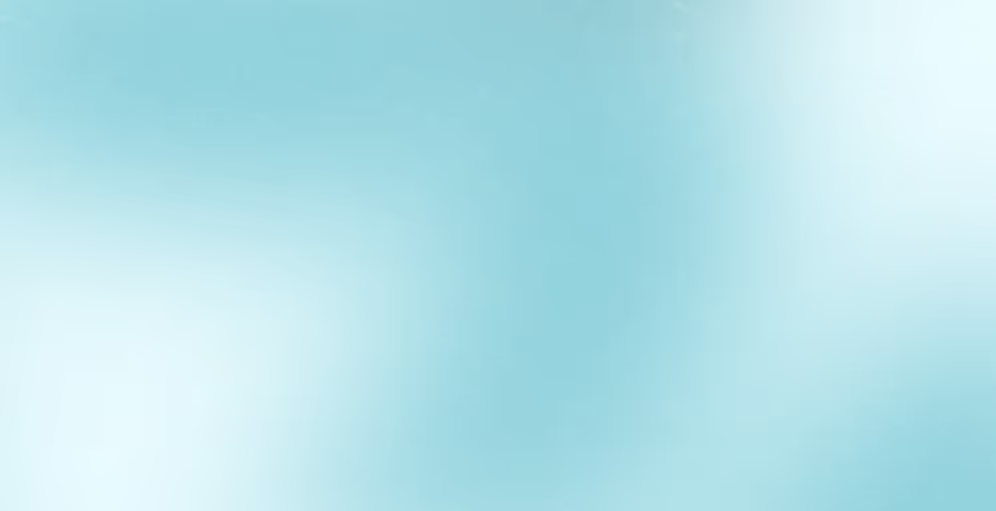
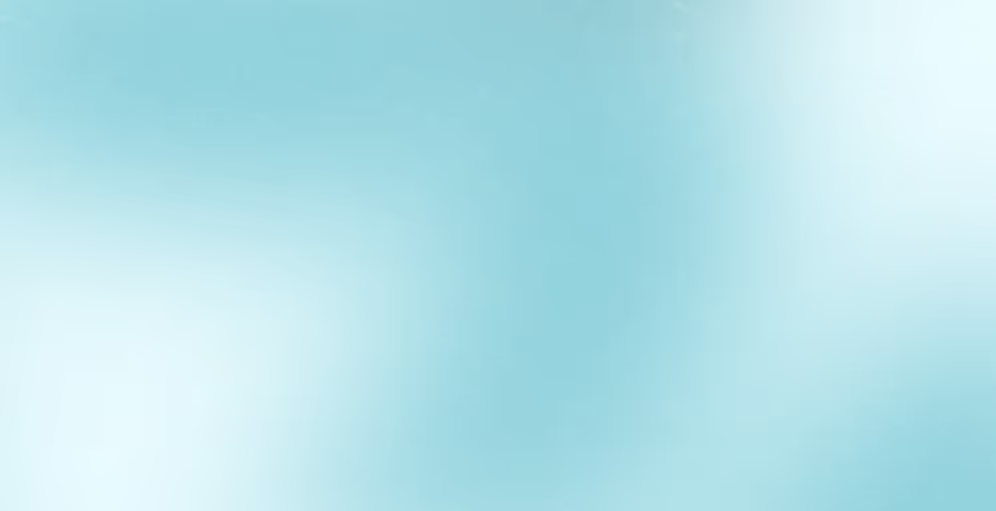
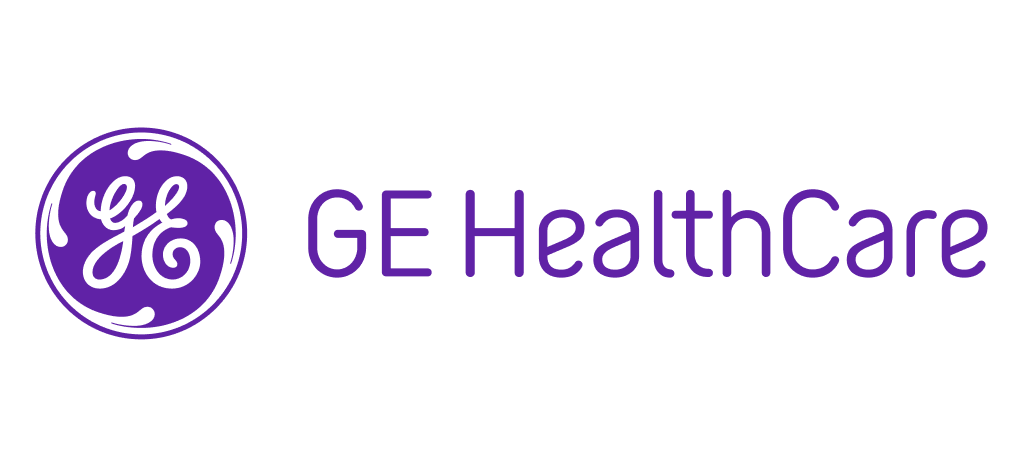
Reduction in model deployment time – from six months to two weeks.
Increased data scientist efficiency – streamlined AI model deployment.
Scalability with reduced overhead – integration of models with minimal custom code.
GE Healthcare, renowned for its leadership in medical imaging and healthcare solutions, continues to push the boundaries of AI and machine learning. At the forefront of this innovation is Selvan Senthivel, Chief Technologist at GE Healthcare, who plays a key role in driving AI initiatives across the company. His team is responsible for developing and deploying advanced machine learning models that enhance medical imaging processes, from MRIs and CT scans to other critical diagnostic tools.
GE’s use of AI spans across various departments, with a particular focus on improving post-processing workflows for medical images. The aim is to create scalable, efficient systems that not only process large volumes of medical data but also integrate seamlessly into highly regulated healthcare environments.
Challenges at GE Healthcare
Before adopting Metaflow, GE Healthcare faced significant bottlenecks in deploying machine learning models at scale. The process of moving a model from development to production was slow and often took up to six months. This was largely due to the limitations of their legacy systems, which required extensive manual configuration for each model.
Deploying large models, such as those used for MR scanning or synthetic CT generation from X-rays and MRIs, was particularly challenging. These models often required significant computing power and careful orchestration to manage the workloads efficiently. In addition, GE’s inferencing services were difficult to use, with each model needing to be packaged and customized for production, adding more time to an already lengthy process.
GE also had to navigate strict regulatory requirements, which made the deployment of AI models even more complicated. Each model had to be thoroughly vetted and meet compliance standards before being released into production, further delaying progress.
The Search for a Solution
Faced with these challenges, Selvan began exploring solutions that would streamline GE’s machine learning processes. His prior experience with Metaflow, an open-source framework he had used while working on bioinformatics pipelines at AWS, made him confident that it could address GE’s specific needs. Metaflow’s ability to transition easily from local development to full-scale production was a key factor in its selection. At GE, the data science team needed a tool that would allow them to quickly prototype models on their local machines and then scale them seamlessly to AWS, without needing to rewrite code or significantly alter their workflows.
Another major advantage of Metaflow was its Python-native design, which made it easy for the entire team to adopt. GE’s data science team is diverse, with members ranging from cutting-edge AI experts to engineers more comfortable with traditional coding methods. Metaflow’s user-friendly approach helped lower the barrier to entry, ensuring that even those less familiar with machine learning could quickly get up to speed. Cost-efficiency was also a critical consideration. Deploying large imaging models required substantial GPU resources, and running these models constantly would have been prohibitively expensive. Metaflow’s infrastructure allowed GE to deploy models only when needed, minimizing costs while maintaining high performance.
Results After Implementing Metaflow
Once Metaflow was implemented, the impact on GE Healthcare’s machine learning processes was immediate and profound. The time it took to deploy models into production dropped from six months to just two weeks. This acceleration was transformative, particularly in the healthcare industry, where the speed at which AI models can be deployed can directly influence patient outcomes. The ability to scale models quickly and efficiently was another significant benefit. Metaflow’s integration with AWS allowed GE to process large volumes of imaging data without overwhelming their systems. Complex imaging models, like those used for post-processing CT scans and MRIs, could be deployed and scaled rapidly, improving the speed at which clinicians received enhanced imaging results.
Metaflow also simplified workflow management for GE’s data science team. Previously, setting up workflows for large models required manual configuration of CPU and GPU resources, which was both time-consuming and error-prone. With Metaflow, these tasks were automated. The team could now define how much computing power a model needed with simple configurations, streamlining the entire process.
In addition to improving workflow efficiency, Metaflow played a key role in reducing operational costs. GE could now run resource-intensive models only when necessary, bringing them online during specific times to optimize usage and then shutting them down to conserve resources. This approach not only reduced costs but also made it easier to manage large-scale tasks such as image segmentation and synthetic imaging generation. Metaflow’s integration with Ray clusters also enabled GE to introduce predictive workflows and active learning loops, which helped forecast hospital operations such as bed occupancy and emergency department traffic. These predictive capabilities allowed hospitals to allocate resources more effectively, improving both operational efficiency and patient care.
Summary of Success
The adoption of Metaflow at GE Healthcare led to a 66% reduction in the time it took to deploy machine learning models, from six months to just two weeks. This speed allowed GE to push innovative imaging models into production faster, significantly improving the company’s ability to deliver timely solutions in the medical field. Additionally, the platform’s scalability enabled GE to process vast amounts of imaging data without sacrificing efficiency or accuracy.
Metaflow’s simplicity also made it easier for GE’s diverse data science team to adopt and leverage its capabilities. By abstracting away much of the complexity of scaling and infrastructure management, Metaflow allowed the team to focus on developing cutting-edge models and delivering faster results. Although regulatory hurdles mean that some models have not yet been deployed externally, GE has already seen the benefits of Metaflow internally. Inferences are being made faster, and the streamlined deployment process is helping GE stay ahead in the highly competitive field of medical imaging. The system is also being used for real-time hospital forecasting, improving operations across several hospitals.
Looking forward, GE Healthcare plans to further expand its use of Metaflow across different departments. One key area of focus is oncology, where advanced machine learning models could play a vital role in identifying treatment options and clinical trials for patients. The company is also exploring the use of Metaflow for their advanced imaging workstations, which would allow clinicians to access enhanced imaging insights in real-time.
As GE continues to push the boundaries of AI in healthcare, Metaflow is set to become an even more integral part of their technology stack. By reducing deployment times, improving scalability, and lowering operational costs, Metaflow is enabling GE to maintain its leadership position in the healthcare industry. Metaflow’s ongoing role at GE Healthcare underscores the transformative power of this framework in complex, real-world environments. Its ability to simplify workflows, scale seamlessly, and handle resource-intensive models is critical to the company’s future success.
Start building today
Join our office hours for a live demo! Whether you're curious about Outerbounds or have specific questions - nothing is off limits.
.png)