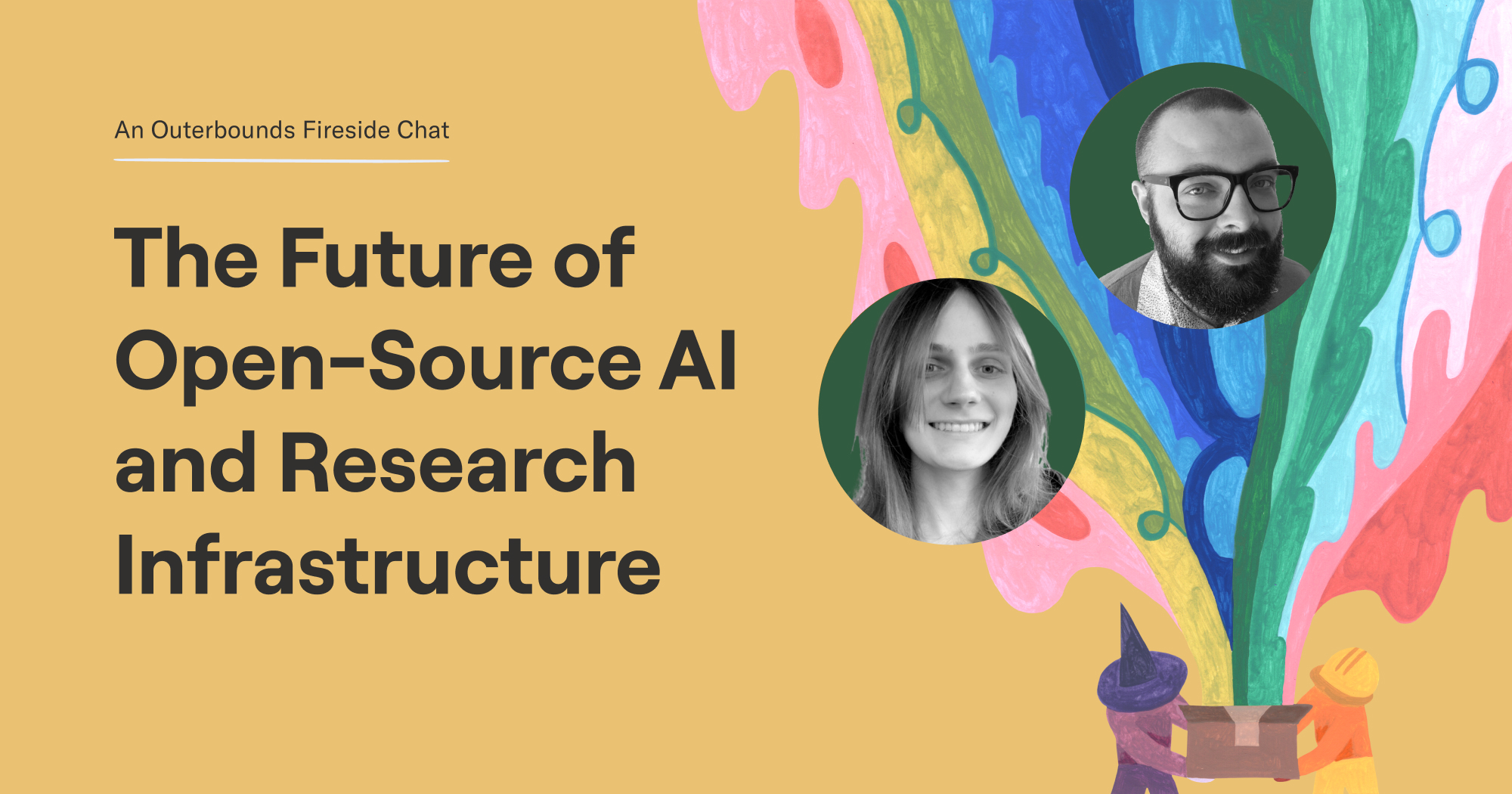
Unlocking the Future of Open-Source AI: A Fireside Chat with Hailey Schoelkopf
We love to dive deep into the conversations shaping the future of AI, and our recent Fireside Chat was no exception. Hosted by Hugo Bowne-Anderson, this event featured Hailey Schoelkopf, a prominent researcher from EleutherAI, who shared invaluable insights into the evolving world of open-source AI, the challenges and benefits of research infrastructure, and the importance of building accessible tools for AI research. Here’s a recap of the key points and thought-provoking moments from the discussion.
EleutherAI: From Grassroots to Leading AI Research
Hailey kicked off the conversation by reflecting on EleutherAI’s journey from a group of machine learning enthusiasts on Discord to a nonprofit leader in AI research. “We started as a grassroots community, with people volunteering on the side,” she said. "It began with a simple idea: after OpenAI published the GPT-2 paper, we asked ourselves, ‘Can we replicate that?’" What started as casual conversations on Discord led to EleutherAI’s landmark contributions like GPT-Neo and GPT-J, which are now widely used open-source models.
This evolution from informal collaboration to a formal nonprofit is a powerful story of how open-source communities can push AI innovation. “It’s been incredible to see the community grow and contribute to projects like the LM Evaluation Harness and GPT-NeoX,” Hailey remarked. “It’s also a testament to the value of building in the open and welcoming collaboration from anyone with a passion for AI.”
Open-Source vs. Open-Weight: The Crucial Difference
One of the core themes of the chat was the distinction between open-source AI tools and open-weight models. Hailey stressed the importance of maintaining both. "Open-source is about providing the tools and code for anyone to use, but open-weight refers to the actual pre-trained models available for research and development." This distinction is critical because open-weight models like those developed by EleutherAI allow researchers to explore and improve upon existing AI systems.
Hailey also highlighted the need for transparency, particularly when it comes to training data and evaluation metrics. She explained, "Without access to open-weight models and clear benchmarks, the research community would struggle to verify results or understand how models are truly performing." This brings us to one of the most important aspects of AI development: evaluation.
The Importance of Evaluation: More Than Just Metrics
As Hailey pointed out, evaluation is not just about performance metrics but understanding the context in which models operate. “Evaluation tools like the LM Evaluation Harness are critical for measuring how well models perform on specific tasks, but also for ensuring they are aligned with the values and needs of the communities they serve,” she explained.
Hailey discussed EleutherAI’s LM Evaluation Harness, which plays a crucial role in providing standardized, reproducible evaluations for large language models. "It’s the backbone for many open AI leaderboards, including Hugging Face’s Open LLM leaderboard," she noted. The harness helps ensure that models are evaluated on a level playing field, making it easier to understand their strengths and limitations.
But as Hailey emphasized, evaluation goes beyond numbers: "The challenge isn’t just achieving high performance on a benchmark; it’s understanding why a model performs the way it does. Are we training it on the right data? Is it biased? These are the questions that need more attention.”
Multimodal AI and Localized Models: The Next Frontier
Hailey also touched on the exciting developments in multimodal AI—systems that can handle more than just text, like images and video. "With the release of models like Meta’s LLaMA 3 and others, we’re seeing a shift toward models that aren’t just general-purpose but can be highly specialized to serve specific needs," she explained. This includes localized models, which cater to specific communities and languages.
EleutherAI has pioneered efforts to create models that serve underrepresented languages and communities. The Polyglot Project is one such initiative, where volunteers helped collect data and train models in different languages. “It’s all about making AI accessible to everyone, not just English speakers or users of major tech platforms,” Hailey said.
The Role of Nonprofits in AI Research
One of the most interesting parts of the conversation revolved around the unique position of nonprofits like EleutherAI in the AI ecosystem. Unlike academia or industry, nonprofits have the freedom to focus on open research that pushes the field forward. “We’re able to work on infrastructure and tools that wouldn’t necessarily be prioritized in a commercial setting,” Hailey explained. This includes creating benchmarks, tools, and datasets that help democratize access to cutting-edge AI technologies.
Building for the Future
As the discussion wrapped up, Hailey offered some reflections on the future of open-source AI and research infrastructure. “We’re seeing more and more players enter the space, and that’s great,” she said. "But we need to keep the focus on transparency and accessibility. AI is only as powerful as the communities that can use it, and that means building tools and models that are open, understandable, and adaptable to a wide range of needs."
Overall, the fireside chat was a fascinating deep dive into the current state and future of AI research, highlighting the importance of open-source contributions and the need for robust, transparent evaluation. Hailey’s insights not only shed light on EleutherAI’s groundbreaking work but also underscored the critical role that open-source communities play in shaping the future of AI.
If you missed the event, be sure to check out the full recording above or on our YouTube channel. Stay tuned for more exciting conversations in our Fireside Chat series!
Start building today
Join our office hours for a live demo! Whether you're curious about Outerbounds or have specific questions - nothing is off limits.