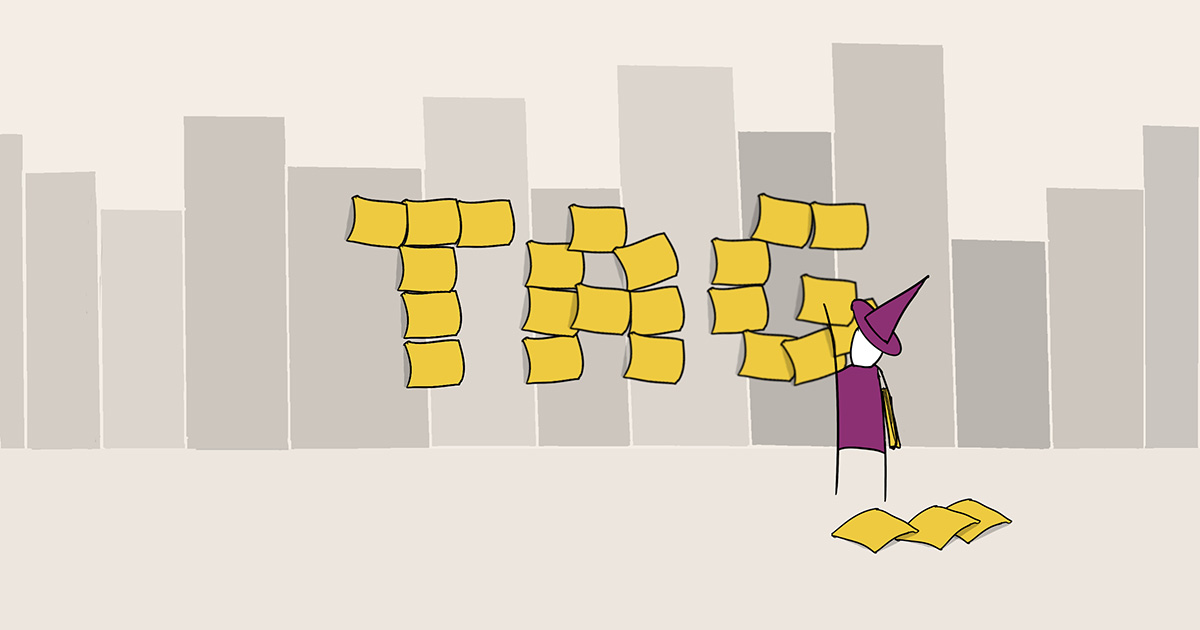
5 Ways to Use the New Metaflow Tags
Metaflow has supported a limited form of tagging via the run --tag
option since its initial open-source release. Thus far it hasn't been possible to mutate tags after a run has completed, which has limited the usefulness of tagging.
We are excited to announce that this restriction has been lifted in the latest Metaflow release 2.7.0 🎉 You are now able to mutate tags freely, which opens up a vast landscape of new use cases for tagging. This article features five such prototypical use cases which have proven to work well at Netflix that has actively leveraged mutable tags for years.
To benefit from mutable tags, make sure your Metaflow version is at least 2.7.0. If you use the metadata service, upgrade the service to the latest version as well. No database migrations are required.
Basic Tagging
Let's start with a basic use case that is familiar from many other tools: Manually attaching tags as human-readable annotations to objects, in our case to Metaflow runs. In this case, tags are used to organize, find, and share results.
In the illustrations below, gray boxes refer to Metaflow runs, 👀 to people taking actions, ⚙️ to production systems that work without human supervision, and yellow labels to tags.

Here's the idea:
- Run Metaflow runs as usual.
- Inspect results using
@card
, notebooks, and Metaflow GUI. - Use the new
tag add
command to attach tags to runs based on the analysis.
See the steps in action:
Programmatic Tagging
Tags allow you to express opinions about results of your and your colleagues' work, and, importantly, change those assessments at any time. In contrast to runs and artifacts that represent immutable facts (history shouldn't be rewritten), the way how you interpret those facts may change over time, which is reflected in tags.
Tagging doesn't have to happen manually. This example shows how to use the tagging methods in the Client API to attach tags to runs automatically. Later, after you have done deeper analysis of the results, you can remove inaccurate tags either via the same API or with the tag remove
command on the command line.

In the video below, we use a code snippet like this
from metaflow import Flow, current
run = Flow(current.flow_name)[current.run_id]
run.add_tag('promising_model')
to attach a tag, promising_model
, to the currently executing run programmatically. After we have iterated on the model for a while, we analyze the promising models in a notebook and use the Run.remove_tag
call to remove the tag from runs that don't deserve the label after a deeper investigation.
Take a look:
Tags and Namespaces
Tags are not only for eyeballing. Internally, Metaflow uses tags to define namespaces. Namespaces are a lightweight way to define boundaries around projects, helping you avoid interfering with colleagues' projects inadvertently, and making it easier to organize your experiments, as illustrated by the following example.

It is common to have a project that spans multiple flows. In cases like this, we often want to fetch results of a flow from another flow. Using an absolute reference like Run('HelloFlow/32')
has limited utility, since new runs get produced constantly and fresh results are typically preferred. A better solution is to use a relative reference like Flow('HelloFlow').latest_run
to refer to another flow.
However, when we say latest_run
- what Run
does it refer to exactly? My latest run, yours, or something else? There is not a single correct answer. To make it possible to frame the answer based on your specific situation, Metaflow returns the latest run in the currently chosen namespace. As namespaces are just sets of runs that share a tag, tagging can be used creatively to organize work across people and projects.
This example walks through a scenario where Anne and Will work together: Anne is responsible for a feature transformation flow and Will for modeling. They experiment and iterate on their flows independently and use tagging to coordinate sharing of results.
Tags in CI/CD
Tags come in handy in production use cases too. In this example, we show how to leverage tags in the context of CI/CD, specifically GitHub Actions.
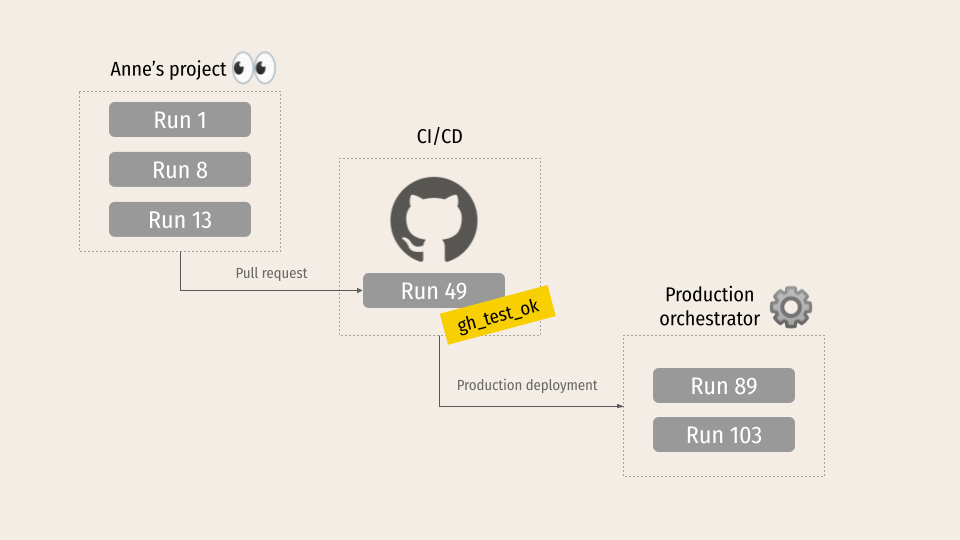
The video demonstrates the following steps:
- The data scientist runs experiments locally as usual.
- When they are happy with the results, they prepare a pull request.
- A pull request triggers a GitHub Action which executes the new flow version as a part of the test suite. Small-scale tests can be run with worker instances provided by the CI/CD system while larger tests can be farmed out to the cloud as usual with Metaflow.
- If tests are successful, the action tags the test run with a tag,
gh_test_ok
, either using the Client API or theadd tag
CLI. - The
gh_test_ok
tags can be used to observe the status of PRs in Metaflow UI and to trigger deployments to production systems.
You can set up a GitOps workflow like this easily at home:
Tags and Continuous Training
This may be one the most impactful use cases for tagging: Consider a production model that should update, say, monthly. Instead of training the model monthly, or triggering retraining reactively based on shifts in data, a more robust pattern is to retrain the model continuously, e.g. daily. Similar to the benefits of Continuous Delivery in software engineering, this pattern minimizes surprises between trainings which are caused by changes in code, data, the execution environment, and human errors.

Metaflow makes it easy to schedule workflows that retrain the model without any human supervision. When it is time to decide whether a new model should be promoted to production, the data scientist can evaluate latest model candidates using @card
, notebooks, Metaflow UI, or even other automated workflows. If the model passes production gates, it is tagged with a tag production
, triggering an actual deployment to e.g. a model hosting environment.
See how we use the pattern with AWS Step Functions:
Conclusion
It is beneficial to allow people to change their minds about many things, including how they view a Metaflow run. This is now possible with mutable tags, which makes them a foundational building block for various workflows that involve a human in the loop. Hopefully these five use cases inspire you to design and deploy patterns that work well for your needs.
We'd love to hear from you and learn about your patterns, use cases, and pain points. Don't be a stranger - join the Metaflow Slack today!
Start building today
Join our office hours for a live demo! Whether you're curious about Outerbounds or have specific questions - nothing is off limits.
.png)