Accelerating Cancer Treatment with Scalable Machine Learning at Artera

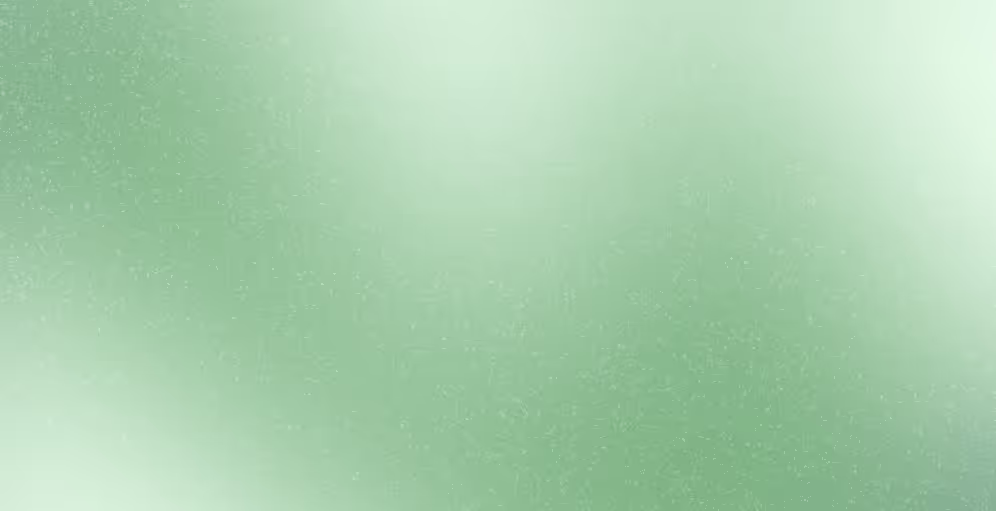

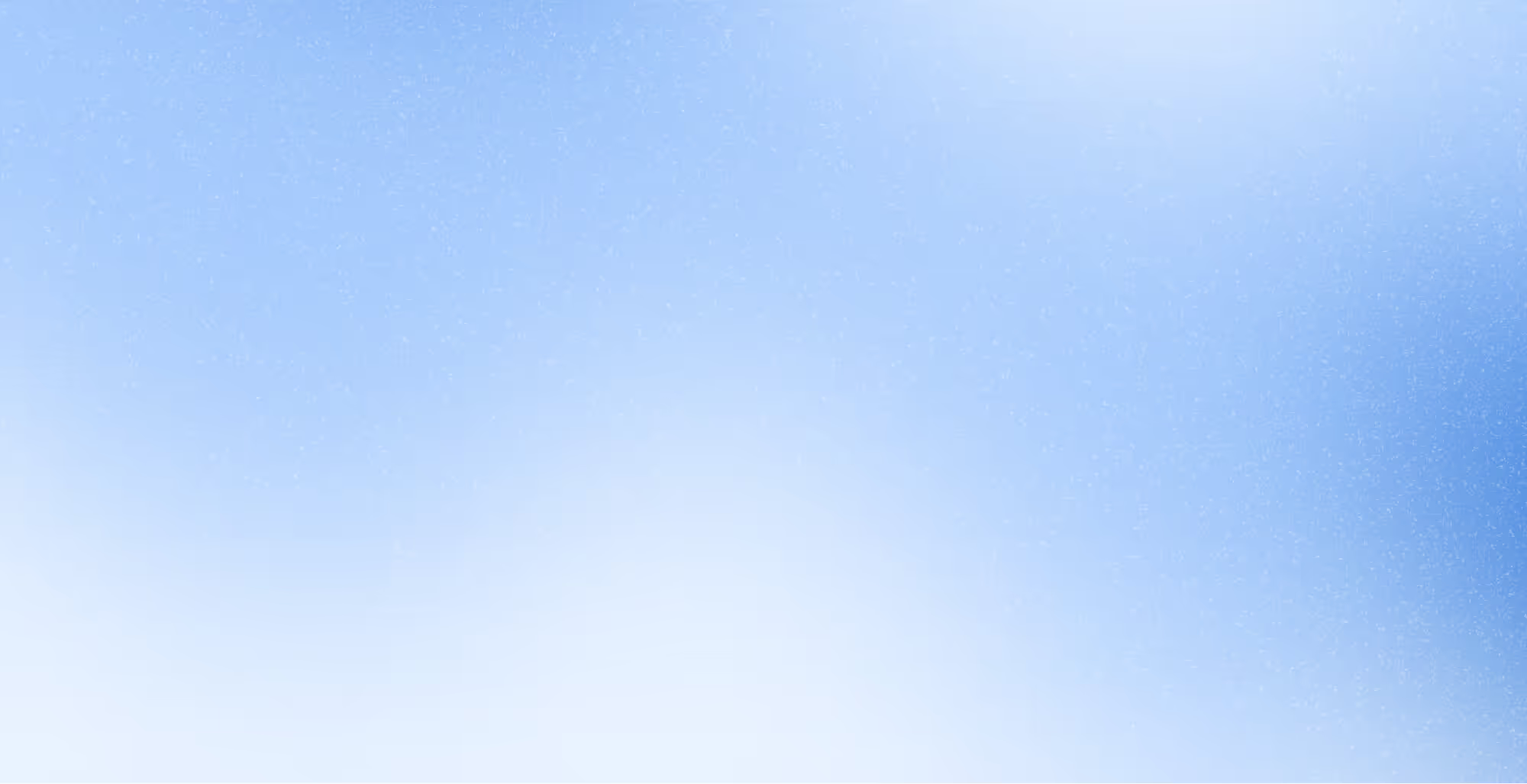
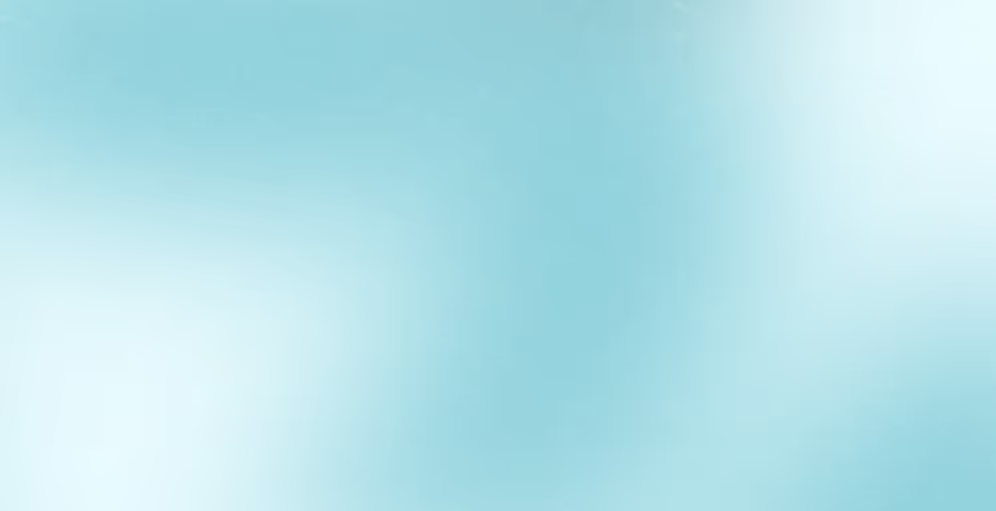
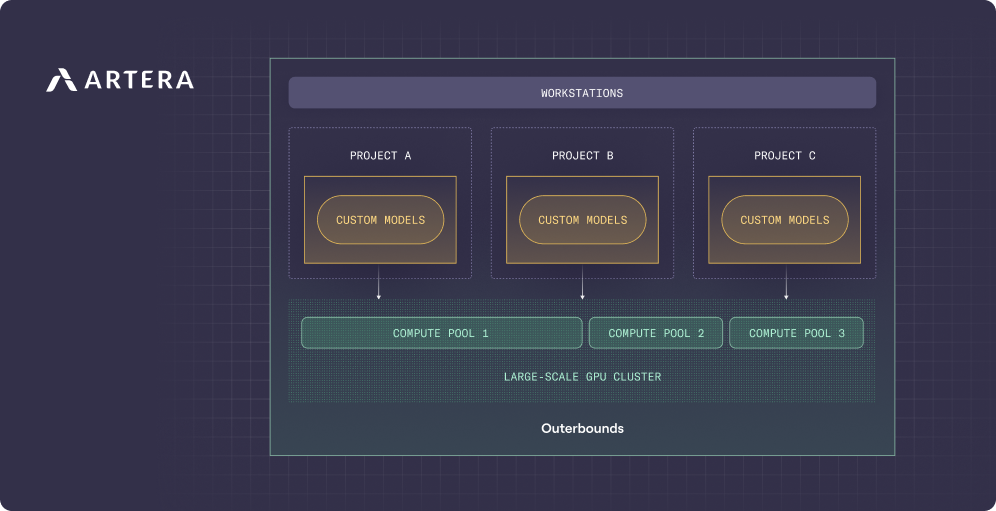

Blockers reduced from 1-2 per quarter to zero after Outerbounds adoption.
Reduced onboarding time for machine learning engineers from weeks to one week.
Increase in model autonomy: data scientists can now deploy models independently.
Artera, recently honored as one of Time.com's Best Inventions of 2024, is a cutting-edge digital pathology company that improves clinical cancer outcomes using machine learning, by providing tools to help doctors make better treatment decisions. Their tests analyze pathology images to give patients more precise risk scores for cancer metastasis, aiding in customized treatment plans. At the forefront of this effort is Akinori Mitani, an AI Platform Manager, who joined Artera after working at Google Health. His transition to Artera reflected his desire to tackle larger data problems in pathology rather than the more limited scope of medical image analysis he encountered in retinal imaging.
"We’re helping doctors by using machine learning models to analyze pathology images and provide risk scores that are more detailed than traditional methods,” Akinori explained, underscoring Artera’s mission to use AI to positively impact cancer treatment.
Initial Challenges: Infrastructure Blockers and Chaos
Upon joining Artera, Akinori quickly discovered that the company’s infrastructure lacked the robustness necessary for efficient machine learning workflows. Compared to his experience at Google, where infrastructure management was seamless and handled by dedicated teams, Artera’s infrastructure was in a state of disarray. Machine learning engineers were operating on AWS EC2 instances, manually setting up environments and configurations, which led to scattered resources and inefficiencies.
"It was chaotic—people were logging into individual EC2 instances and running things manually. It took a long time just to figure out how to set up parallel jobs,” Akinori recalled.
The company was trying to build scalable systems for their machine learning models, but regularly encountered hurdles. This included challenges in managing massive pathology images, often hundreds of thousands of pixels wide, making them computationally expensive to process. Without a strong infrastructure, this problem led to frequent delays and even job failures.
To cope with this, Artera experimented with different internal solutions. They initially relied on TorchX, a job scheduling system wrapped around Kubernetes, but it was inefficient. Later, they moved to Fireworks AIH, which showed some improvement but was still prone to frequent blockers. The team would hit capacity limits, causing jobs to fail or hang, and when this happened, multiple engineers would be affected. These issues occurred as often as once or twice per quarter, causing significant delays in project timelines.
"It wasn’t just about the time spent fixing the problem; it was the uncertainty it created," Akinori said. "When something went wrong, you didn’t always know what caused it, and that was frustrating."
One of the main challenges was how to process large-scale pathology images, which could not be handled efficiently with the existing infrastructure. Preprocessing these images was slow, and the system frequently ran out of compute resources, making tasks like hyperparameter optimization for model training frustratingly slow. This bottleneck became a clear blocker in scaling the machine learning operations that were critical to Artera’s mission.
Searching for the Right Solution: Outerbounds
In the search for a better infrastructure solution, Artera explored several SaaS platforms, evaluating them against stringent criteria. The company needed a tool that could not only handle the complex compute jobs involved in processing high-resolution pathology images but also scale seamlessly and support local development with GPU access. Additionally, Artera, as a healthcare company, had strict data privacy and regulatory concerns, including HIPAA compliance.
Outerbounds surfaced as the top contender. It provided several critical features that aligned with Artera’s needs, such as scalable infrastructure, simplified job scheduling, and strong support for local GPU-based development. One standout feature was the ability to spin up and down GPU workstations seamlessly, which allowed engineers to prototype and test models on local machines and then scale to larger environments when necessary.
"Outerbounds’ workstation support stood out immediately," said Akinori. "We could finally run jobs locally with GPUs and seamlessly scale to hundreds of machines for larger jobs. The UI was stable, and the product was well-designed overall."
Another significant feature was Outerbounds’ support for AWS EFS (Elastic File System), which addressed one of Artera’s most pressing concerns: the ability to support quick model development iterations. With vast amounts of data being used, Artera required a storage solution that could easily integrate with their compute workflows, and EFS became a crucial part of that system. Although Outerbounds didn’t initially support EFS, the team quickly promised that it would, and within a few weeks, that support was delivered.
"One thing that really impressed us was how quickly the Outerbounds team implemented EFS support after we raised it as a blocker. That flexibility and responsiveness sealed the deal for us," Akinori added.
Streamlined Workflow, Faster Onboarding, and Zero Blockers
After implementing Outerbounds, the impact on Artera’s workflow was immediate and significant. Tasks that previously took days or weeks were now completed in hours, thanks to the ability to run parallel processes across a cluster of machines. Preprocessing the massive pathology images became much easier, and tasks like hyperparameter optimization, which had previously been a bottleneck, were now routine.
"Before Outerbounds, processing a large set of pathology images or running a hyperparameter search could take days. Now we can scale across hundreds of machines and get it done in hours. That’s a game-changer for us," Akinori explained.
A key result was the complete elimination of infrastructure blockers. Before Outerbounds, engineers would frequently encounter issues where jobs would fail to start, hang, or misbehave due to underlying infrastructure limits or misconfigurations. These issues happened one or two times per quarter, disrupting the entire team. Since adopting Outerbounds, these blockers have disappeared entirely, leading to a 100% reduction in infrastructure-related disruptions.
"We used to get blocked by infrastructure problems every quarter, but since moving to Outerbounds, that number has dropped to zero," said Akinori. "We’re no longer wasting time troubleshooting infrastructure—we’re focused on developing better models."
In addition to reducing infrastructure issues, Outerbounds also streamlined the onboarding process for new machine learning engineers. Previously, onboarding could take weeks, as new hires had to manually configure EC2 instances and navigate complex role management systems on AWS. With Outerbounds, the entire team of ten machine learning engineers was onboarded in just one week, thanks to a simplified, consistent environment that made it easy to access the necessary resources.
"We onboarded ten engineers in about a week. Before, it could take much longer, especially with all the manual configuration required in the old system," Akinori recalled.
With Outerbounds now firmly integrated into their workflow, Artera is looking ahead to further optimize and streamline their machine learning operations. One area of focus is automating more of the preprocessing and inference tasks, allowing their teams to experiment with new models and datasets more easily.
"Now that we have the right infrastructure in place, we’re focused on speeding up the model development cycle even further," Akinori noted. "We want to make it easier for our teams to kick off experiments and scale them quickly."
Outerbounds has transformed how Artera approaches machine learning. The platform’s stability, scalability, and support for large-scale parallel processing have enabled the team to focus on what truly matters: improving cancer treatment through cutting-edge machine learning models. By eliminating infrastructure blockers, speeding up onboarding, and providing flexible GPU workstations, Outerbounds has become an indispensable part of Artera’s machine learning operations.
In Akinori’s words, "Outerbounds has taken the pain out of infrastructure management and allowed us to focus on developing models that can make a real difference in patients’ lives. It’s been a huge success."
As Artera continues to grow, the company plans to deepen its use of Outerbounds, leveraging its full capabilities to handle even larger datasets and more complex machine learning workflows.
Start building today
Join our office hours for a live demo! Whether you're curious about Outerbounds or have specific questions - nothing is off limits.
.png)